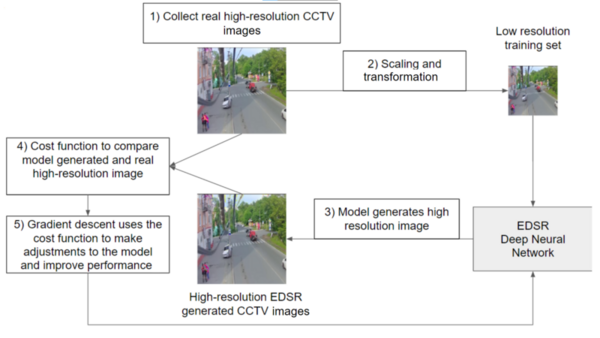
In this study, the authors hypothesized that closed-circuit television images could be stored with improved resolution by using enhanced deep residual (EDSR) networks.
Read More...Deep residual neural networks for increasing the resolution of CCTV images
In this study, the authors hypothesized that closed-circuit television images could be stored with improved resolution by using enhanced deep residual (EDSR) networks.
Read More...Enhancing marine debris identification with convolutional neural networks
Plastic pollution in the ocean is a major global concern. Remotely Operated Vehicles (ROVs) have promise for removing debris from the ocean, but more research is needed to achieve full effectiveness of the ROV technology. Wahlig and Gonzales tackle this issue by developing a deep learning model to distinguish trash from the environment in ROV images.
Read More...Automated classification of nebulae using deep learning & machine learning for enhanced discovery
There are believed to be ~20,000 nebulae in the Milky Way Galaxy. However, humans have only cataloged ~1,800 of them even though we have gathered 1.3 million nebula images. Classification of nebulae is important as it helps scientists understand the chemical composition of a nebula which in turn helps them understand the material of the original star. Our research on nebulae classification aims to make the process of classifying new nebulae faster and more accurate using a hybrid of deep learning and machine learning techniques.
Read More...Building deep neural networks to detect candy from photos and estimate nutrient portfolio
The authors use pictures of candy wrappers and neural networks to improve nutritional accuracy of diet-tracking apps.
Read More...The Role of a Mask - Understanding the Performance of Deep Neural Networks to Detect, Segment, and Extract Cellular Nuclei from Microscopy Images
Cell segmentation is the task of identifying cell nuclei instances in fluorescence microscopy images. The goal of this paper is to benchmark the performance of representative deep learning techniques for cell nuclei segmentation using standard datasets and common evaluation criteria. This research establishes an important baseline for cell nuclei segmentation, enabling researchers to continually refine and deploy neural models for real-world clinical applications.
Read More...A novel approach for early detection of Alzheimer’s disease using deep neural networks with magnetic resonance imaging
In the battle against Alzheimer's disease, early detection is critical to mitigating symptoms in patients. Here, the authors use a collection of MRI scans, layering with deep learning computer modeling, to investigate early stages of AD which can be hard to catch by human eye. Their model is successful, able to outperform previous models, and detected regions of interest in the brain for further consideration.
Read More...Antibiotic Residues Detected in Commercial Cow’s Milk
Antibiotics are oftentimes used to treat mastitis (infection of the mammary gland) in dairy cows. Regulations require that milk from these cows be discarded until the infection has cleared and antibiotic residues are no longer detectable in the cow's milk. These regulations are in place to protect consumers and to help prevent the rise of antibiotic resistant bacteria. In this study, the authors test milk samples from 10 milk suppliers in the Greensboro, NC to see if they contain detectable levels of antibiotic residues.
Read More...Determining the best convolutional neural network for identifying tuberculosis and pneumonia in chest x-rays
To best identify tuberculosis and pneumonia diagnoses in chest x-rays, the authors compare different deep learning convolution neural networks.
Read More...Evaluating the clinical applicability of neural networks for meningioma tumor segmentation on 3D MRI
Authors emphasize the challenges of manual tumor segmentation and the potential of deep learning models to enhance accuracy by automatically analyzing MRI scans.
Read More...Tomato disease identification with shallow convolutional neural networks
Plant diseases can cause up to 50% crop yield loss for the popular tomato plant. A mobile device-based method to identify diseases from photos of symptomatic leaves via computer vision can be more effective due to its convenience and accessibility. To enable a practical mobile solution, a “shallow” convolutional neural networks (CNNs) with few layers, and thus low computational requirement but with high accuracy similar to the deep CNNs is needed. In this work, we explored if such a model was possible.
Read More...Search articles by title, author name, or tags