Enhancing marine debris identification with convolutional neural networks
(1) Falmouth High School, (2) Radcliffe Department of Medicine, University of Oxford
https://doi.org/10.59720/23-150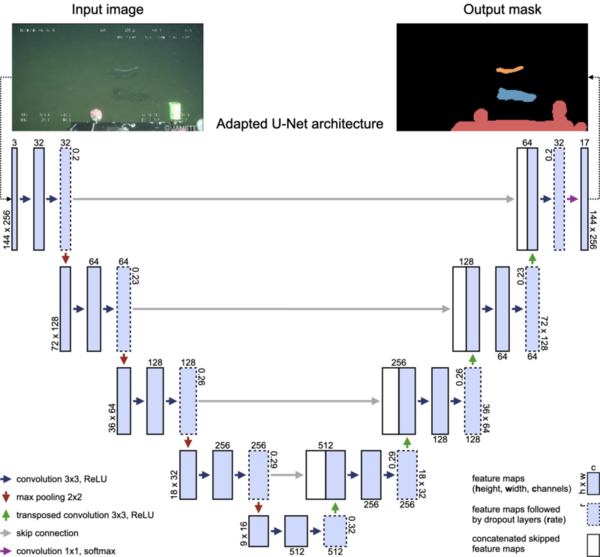
Oceanic debris, predominantly plastic, inflicts catastrophic damage on marine ecosystems, threatens aquatic life through entanglement, strangulation, and starvation, and increasingly accumulates as microplastics within the tissues of marine species consumed by humans. Assessing and mitigating this crisis is complicated by the wide-ranging distribution of waste by ocean currents to remote and deep-sea locations. In this study, we developed a deep learning model to discern and identify components of images captured by an underwater remotely operated vehicle (ROV). We hypothesized that the model would achieve an 80% accuracy. We utilized image segmentation, a process that groups or "masks" all pixels associated with a specific object in the image, to recognize and delineate image components (trash, animal, plant, ROV). We trained our model using the established TrashCan 1.0 dataset, which comprises images captured by ROVs in the Sea of Japan. Our model, a convolutional neural network employing U-Net architecture, formulated feature maps for each object within the images, enabling prediction of object classes. During the testing phase, we compared the model-generated object masks against reference masks to establish the Dice Similarity Coefficient (DSC) for each object class (trash = 0.81 ± 0.38, animal = 0.85 ± 0.35, plant = 0.88 ± 0.31, ROV = 0.86 ± 0.29, overall average = 0.84 ± 0.36). The consistent categorization of image components by our model demonstrates its potential as an effective ROV-borne tool capable of identifying and targeting hitherto inaccessible ocean trash. Our study lays a robust foundation for future research and additional applications.
This article has been tagged with: