Tomato disease identification with shallow convolutional neural networks
(1) University High School, Irvine, California
https://doi.org/10.59720/22-171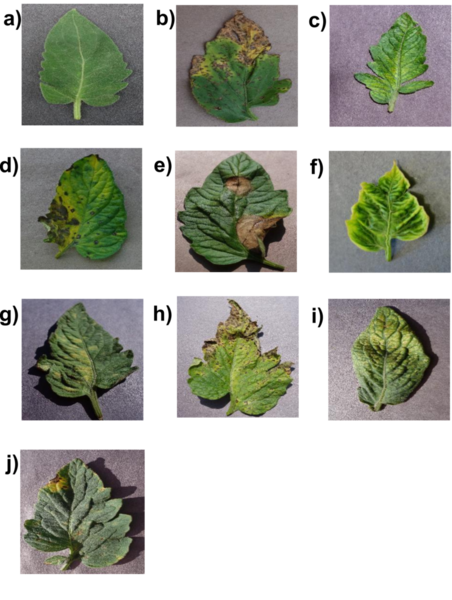
Plant diseases can cause up to 50% crop yield loss for the popular tomato plant. Successful disease management requires precise and timely disease identification methods, but slow and inconsistent diagnoses from human experts or laboratory testing prevents farmers from applying appropriate treatments before the disease takes its toll. As an alternative diagnostic method, a mobile device-based method to identify diseases from photos of symptomatic leaves via computer vision can be more effective due to its convenience and accessibility. Previous research has achieved high accuracy in diagnoses using “deep” convolutional neural networks (CNNs) that have a large number of processing layers and thus a large computational requirement difficult to satisfy on most mobile devices. To enable a practical mobile solution, a “shallow” CNN with few layers, and thus low computational requirement but with high accuracy similar to the deep CNNs is needed. In this work, we explored if such a model was possible. We hypothesized that shallow CNNs would be capable of achieving even higher accuracy than deep CNNs because of shallow CNNs’ relatively low inherent dimensionality reduction, allowing it to preserve more distinguishing features between photos of leaves with different diseases. We compared the performance of a shallow CNN we built against conventional deep CNNs. The shallow CNN outperformed several deep CNNs, confirming our hypothesis. This finding enabled us to develop PlantDoctor, a mobile app that can accurately identify plant diseases via leaf images captured by the phone camera in real-time and suggest appropriate treatments.
This article has been tagged with: