Deep residual neural networks for increasing the resolution of CCTV images
(1) Canyon Crest Academy, San Diego, California, (2) Downing College, University of Cambridge, Cambridge, UK
https://doi.org/10.59720/21-052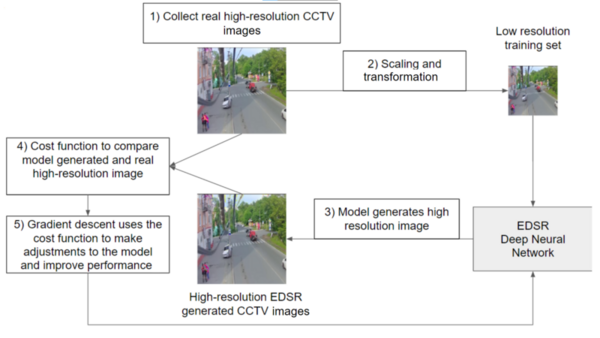
Images from closed-circuit television (CCTV) cameras are often stored with poor resolution due to technical and data storage limitations, reducing the utility of these images. In this study, we propose that informed design and training of an enhanced deep residual network (EDSR) can increase the resolution of CCTV images. This work is the first published application of the EDSR methodology for increasing CCTV image resolution. We utilized a dataset of 986 CCTV images that trained and tested an EDSR for generating high-resolution images from a low-resolution dataset. Implementing an EDSR model, we demonstrate that with 60 layers, performing image super-resolution is extremely effective, producing a peak signal-to-noise ratio value of 76.83 decibels. Furthermore, this technique may be used more generally as a technique for file compression enabling the storage of images at smaller file sizes at low-resolution and the ability to reproduce the same images in high-resolution form.
This article has been tagged with: