Evaluating the clinical applicability of neural networks for meningioma tumor segmentation on 3D MRI
(1) Troy High School, (2) Department of Neurosurgery, Perelman School of Medicine, University of Pennsylvania
https://doi.org/10.59720/23-265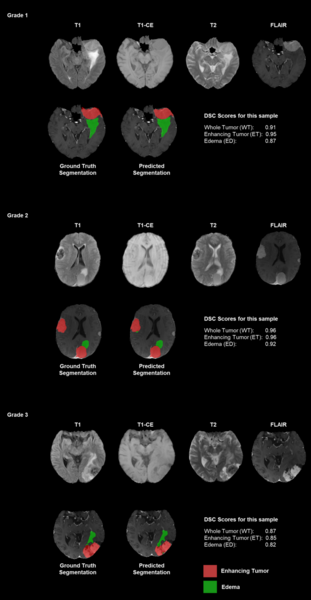
Magnetic resonance imaging (MRI), in both 2D and 3D configurations, serves as the leading non-invasive and non-ionizing technique for the detection and clinical assessment of brain tumors. 3D multiparametric MRIs (mpMRIs) offer enhanced spatial and biological context over other MRIs, which can help clinicians plan tailored treatment and therapy. Presently, surgical intervention remains the primary treatment method for brain tumors, requiring accurate segmentation of tumor tissue on MRI scans. However, manual tumor segmentation and traditional machine learning techniques are labor intensive and subject to observer bias. Deep learning models can provide enhanced accuracy and precision in tumor segmentation due to their ability to automatically extract intricate features and patterns from MRI scans. In this study, MRI scans from 358 meningioma patients were used to train a series of deep learning models to segment the tumor subregions. We hypothesized that a deep learning framework designed for meningioma tumor segmentation and trained utilizing 3D mpMRIs would demonstrate superior segmentation accuracy compared to a framework trained on 2D MRIs or single-sequence 3D MRIs. We compared the model predictions with the ground truth labels using similarity metrics to assess accuracy and clinical applicability. The model trained on 3D mpMRI scans showed reliable performance for meningioma subregion segmentation with a Dice Similarity Coefficient score of 0.91 and a median Sensitivity of 91.38%. It outperformed the other models due to the additional spatial context provided by 3D mpMRIs, suggesting it could be readily used in clinical practice to help guide treatment strategy.
This article has been tagged with: