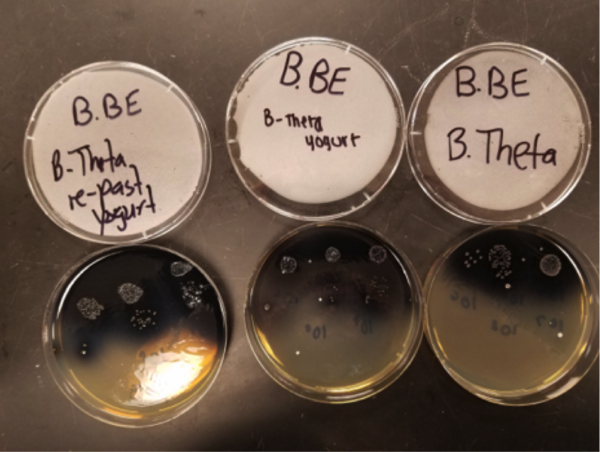
Disruptions to the microbiome, specifically the imbalance in the two major phyla, the Firmicutes and the Bacteroidetes, have been linked to the development of obesity. This study explored whether or not Fage plain total 0% Greek yogurt, which contains live and active bacterial cultures belonging to the Firmicute phylum, could decrease the numbers of Bacteroides thetaiotaomicron, an organism found in the human gut that belongs to the Bacteroidetes phylum.
Read More...