Transfer Learning with Convolutional Neural Network-Based Models for Skin Cancer Classification
(1) Saratoga High School, (2) AIClub
https://doi.org/10.59720/23-204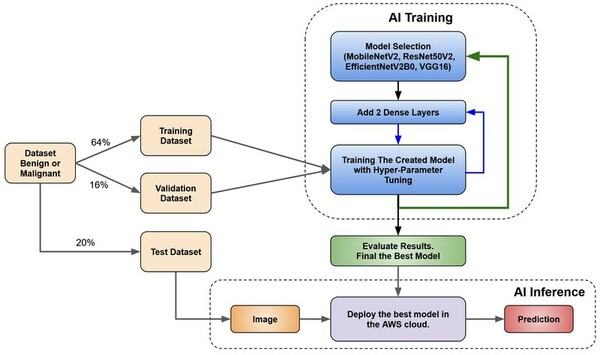
Skin cancer is a common and potentially deadly form of cancer. According to the Skin Cancer Foundation, 1 in 5 Americans will develop skin cancer by the age of 70. If caught early, it can often be treated with minimal surgical intervention and a high likelihood of full recovery. However, diagnosis using current methods requires a physician, is time-consuming, and is expensive. This study’s purpose was to develop an automated approach for early detection for skin cancer. We hypothesized that convolutional neural network-based models using transfer learning could accurately differentiate between benign and malignant moles using natural images of human skin. To test this hypothesis, we developed a skin cancer detection system using four types of deep learning model architectures MobileNetV2, ResNet50V2, EfficientNetV2B0, and VGG16. We tested our models with a publicly available dataset from International Skin Imaging Collaboration. Through training, evaluation, and hyper-parameter tuning across four different models, the best-performing model, VGG16, achieved an Area Under the Curve (AUC) score of 0.95 and a test accuracy of 84.7%. We deployed the model as a publicly available service using Representational State Transfer Application Programming Interface (REST API). Skin images can be submitted to the API endpoint for skin cancer prediction. Our findings suggest that deep learning models can play a vital role in accessible and automated skin cancer screening, empowering healthcare professionals to make informed decisions and potentially improving outcomes for patients with limited access to healthcare resources.
This article has been tagged with: