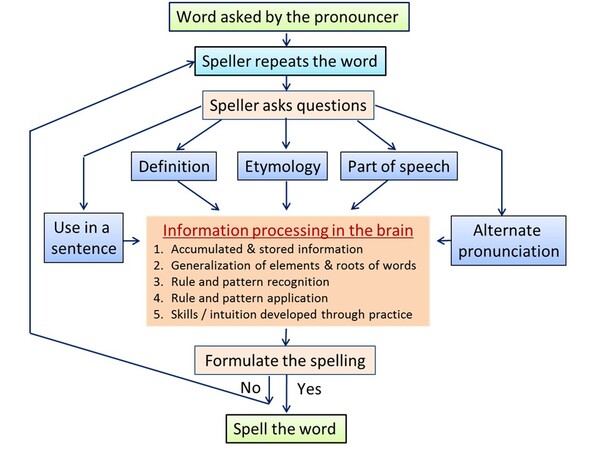
This article investigates the study methodologies, learning strategies, and motives of spelling bee participants. The authors identify several important educational implications of this work.
Read More...Spelling Bee: A Study on the Motivation and Learning Strategies Among Elementary and Junior-High Student Competitors
This article investigates the study methodologies, learning strategies, and motives of spelling bee participants. The authors identify several important educational implications of this work.
Read More...Building a video classifier to improve the accuracy of depth-aware frame interpolation
In this study, the authors share their work on improving the frame rate of videos to reduce data sent to users with both 2D and 3D footage. This work helps improve the experience for both types of footage!
Read More...Using machine learning to develop a global coral bleaching predictor
Coral bleaching is a fatal process that reduces coral diversity, leads to habitat loss for marine organisms, and is a symptom of climate change. This process occurs when corals expel their symbiotic dinoflagellates, algae that photosynthesize within coral tissue providing corals with glucose. Restoration efforts have attempted to repair damaged reefs; however, there are over 360,000 square miles of coral reefs worldwide, making it challenging to target conservation efforts. Thus, predicting the likelihood of bleaching in a certain region would make it easier to allocate resources for conservation efforts. We developed a machine learning model to predict global locations at risk for coral bleaching. Data obtained from the Biological and Chemical Oceanography Data Management Office consisted of various coral bleaching events and the parameters under which the bleaching occurred. Sea surface temperature, sea surface temperature anomalies, longitude, latitude, and coral depth below the surface were the features found to be most correlated to coral bleaching. Thirty-nine machine learning models were tested to determine which one most accurately used the parameters of interest to predict the percentage of corals that would be bleached. A random forest regressor model with an R-squared value of 0.25 and a root mean squared error value of 7.91 was determined to be the best model for predicting coral bleaching. In the end, the random model had a 96% accuracy in predicting the percentage of corals that would be bleached. This prediction system can make it easier for researchers and conservationists to identify coral bleaching hotspots and properly allocate resources to prevent or mitigate bleaching events.
Read More...Comparative singlet oxygen photosensitizer efficiency of berberine, rose bengal, and methylene blue by time course nuclear magnetic resonance (NMR) monitoring of a photochemical 4+2 cycloaddition endoperoxide formation
Berberine, a natural product alkaloid, has been shown to exert biological activity via in situ production of singlet oxygen when photo irradiated. Berberine utilizes singlet oxygen in its putative mechanism of action, wherein it forms an activated complex with DNA and photosensitizes triplet oxygen to singlet oxygen to specifically oxidize guanine residues, thereby halting cell replication and leading to cell death. This has potential application in photodynamic therapy, alongside other such compounds which also act as photosensitizers and produce singlet oxygen in situ. The quantification of singlet oxygen in various photosensitizers, including berberine, is essential for determining their photosensitizer efficiencies. We postulated that the singlet oxygen produced by photoirradiation of berberine would be superior in terms of singlet oxygen production to the aforementioned photosensitizers when irradiated with UV light, but inferior under visible light conditions, due to its strong absorbance of UV wavelengths.
Read More...Effects of Temperature on Hand Sanitizer Efficiency
Here, recognizing the widespread use of hand sanitizers, the authors investigated their effectiveness in relation to storage temperature. They applied hand sanitizer before and after touching a cell phone and used LB-agar plates to monitor the growth of bacteria following this process. They found that 70% ethyl-alcohol-based sanitizers are least effective at temperatures above 107.27 °F and most effective at 96.17 °F.
Read More...Effects of Common Pesticides on Population Size, Motor Function, and Learning Capabilities in Drosophilia melanogaster
In this study, the authors examined the effects of commonly used pesticides (metolachlor, glyphosate, chlorpyrifos, and atrazine) on population size, motor function, and learning in Drosophila melanogaster.
Read More...Pressure and temperature influence the efficacy of metal-organic frameworks for carbon capture and conversion
Metal-organic frameworks (MOFs) are promising new nanomaterials for use in the fight against climate change that can efficiently capture and convert CO2 to other useful carbon products. This research used computational models to determine the reaction conditions under which MOFs can more efficiently capture and convert CO2. In a cost-efficient manner, this analysis tested the hypothesis that pressure and temperature affect the efficacy of carbon capture and conversion, and contribute to understanding the optimal conditions for MOF performance to improve the use of MOFs for controlling greenhouse CO2 emissions.
Read More...Prediction of molecular energy using Coulomb matrix and Graph Neural Network
With molecular energy being an integral element to the study of molecules and molecular interactions, computational methods to determine molecular energy are used for the preservation of time and resources. However, these computational methods have high demand for computer resources, limiting their widespread feasibility. The authors of this study employed machine learning to address this disadvantage, utilizing neural networks trained on different representations of molecules to predict molecular properties without the requirement of computationally-intensive processing. In their findings, the authors determined the Feedforward Neural Network, trained by two separate models, as capable of predicting molecular energy with limited prediction error.
Read More...Open Source RNN designed for text generation is capable of composing music similar to Baroque composers
Recurrent neural networks (RNNs) are useful for text generation since they can generate outputs in the context of previous ones. Baroque music and language are similar, as every word or note exists in context with others, and they both follow strict rules. The authors hypothesized that if we represent music in a text format, an RNN designed to generate language could train on it and create music structurally similar to Bach’s. They found that the music generated by our RNN shared a similar structure with Bach’s music in the input dataset, while Bachbot’s outputs are significantly different from this experiment’s outputs and thus are less similar to Bach’s repertoire compared to our algorithm.
Read More...A study of South Korean international school students: Impact of COVID-19 on anxiety and learning habits
In this study, the authors investigate the effects of the COVID-19 pandemic on South Korean international school students' anxiety, well being and their learning habits.
Read More...Search articles by title, author name, or tags