Prediction of molecular energy using Coulomb matrix and Graph Neural Network
(1) Skyline High School, Sammamish, Washington
https://doi.org/10.59720/21-026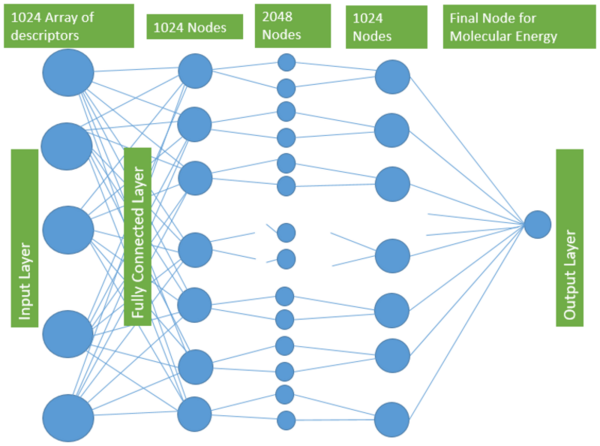
Molecular energy is used to estimate the overall favorability of the formation of a molecule, which can be measured either directly or indirectly. However, both methods require a chemical reaction. Molecular energy has also been investigated computationally. Yet, these calculations require a lot of expensive computer resources. The purpose of this project is to apply machine learning (ML) techniques to create models from known molecular properties. Once the model is created, the molecular properties of new molecules can be predicted quickly. Feedforward Neural Network (FNN) models were trained with two different representations of the molecules. In the first model, the molecules were represented by the Coulombic force between each atom; in the second model, the molecular structure, the bonding between atoms, and the electron configuration of each atom were provided as inputs. Prediction errors of the two models were compared statistically to determine the better representation of the molecule. The FNN network was able to predict the molecular energy with low errors in both models. Though, the model with the Coulomb matrix performed better for molecules that do not contain high electronegative atoms and performed poorly for molecules with three or more fluorine atoms. The effect of fluorine (or other electronegative atoms) on the Coulomb matrix needs to be further investigated.
This article has been tagged with: