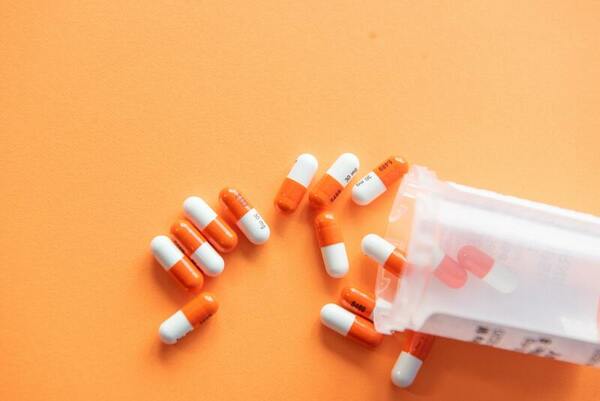
In this study, the authors investigate associations between use of histamine H2 receptor antagonists and suicidal ideation in different age groups.
Read More...Association of depression and suicidal ideation among adults with the use of H2 antagonists
In this study, the authors investigate associations between use of histamine H2 receptor antagonists and suicidal ideation in different age groups.
Read More...Reducing levels of C-Reactive Protein: An eight-week, open-label clinical trial of three oral supplements
In this study, the effects of vitamin C, ginger, or curcumin supplements on C-reactive protein levels in healthy participants are determined in an eight-week open-label trial.
Read More...The Effects of Ocean Acidification on the food location behavior and Locomotion of Pagurus Longicarpus
Increasing levels of atmospheric carbon dioxide is slowly acidifying our oceans. Here the authors test the effects of ocean acidification on the ability of hermit crabs (P. longicarpus) to find food. Though no statistically significant changes in food finding were observed, the data suggest a trend toward different activity.
Read More...Developing “Off the Shelf” Pancreases for Diabetic Patients Using Bacterial and Kombucha Tea Waste
In this study, the authors investigate the suitability of using bacterial cellulose as a scaffold for cell transplants. Interestingly, this cellulose is a can be found in the discard from a symbiotic culture of bacteria and yeast (SCOBY) used to make kombucha.
Read More...A novel calibration algorithm and its effects on heading measurement accuracy of a low-cost magnetometer
Digital compasses are essential in technology that we use in our everyday lives: phones, vehicles, and more. Li and Liu address the accuracy of these devices by presenting a new algorithm for accurately calibrating low-cost magnetometers.
Read More...Collaboration beats heterogeneity: Improving federated learning-based waste classification
Based on the success of deep learning, recent works have attempted to develop a waste classification model using deep neural networks. This work presents federated learning (FL) for a solution, as it allows participants to aid in training the model using their own data. Results showed that with less clients, having a higher participation ratio resulted in less accuracy degradation by the data heterogeneity.
Read More...Reddit v. Wall Street: Why Redditors beat Wall Street at its own game
Here the authors investigated the motivation of a short squeeze of GameStop stock where users of the internet forum Reddit drove a sudden increase in GameStop stock price during the start of 2021. They relied on both qualitative and quantitative analyses where they tracked activity on the r/WallStreetBets subreddit in relation to mentions of GameStop. With these methods they found that while initially the short squeeze was driven by financial motivations, later on emotional motivations became more important. They suggest that social phenomena can be dynamic and evolve necessitating mixed method approaches to study them.
Read More...Examining What Causes Perceived Dissonance in Musical Intervals and the Effect of Timbre on Dissonance
Music is an important part of the day for many of us, but what makes some combinations of sound more pleasant to the ear than others? In this article the authors investigate the role of some characteristics of sound on the perception of a pleasant vs harsh musical note.
Read More...The most efficient position of magnets
Here, the authors investigated the most efficient way to position magnets to hold the most pieces of paper on the surface of a refrigerator. They used a regression model along with an artificial neural network to identify the most efficient positions of four magnets to be at the vertices of a rectangle.
Read More...Artificial Intelligence Networks Towards Learning Without Forgetting
In their paper, Kreiman et al. examined what it takes for an artificial neural network to be able to perform well on a new task without forgetting its previous knowledge. By comparing methods that stop task forgetting, they found that longer training times and maintenance of the most important connections in a particular task while training on a new one helped the neural network maintain its performance on both tasks. The authors hope that this proof-of-principle research will someday contribute to artificial intelligence that better mimics natural human intelligence.
Read More...Search articles by title, author name, or tags