Collaboration beats heterogeneity: Improving federated learning-based waste classification
(1) Seoul Scholars International Math and Science, Seoul, South Korea, (2) SAP Labs Korea, Seoul, South Korea
https://doi.org/10.59720/22-158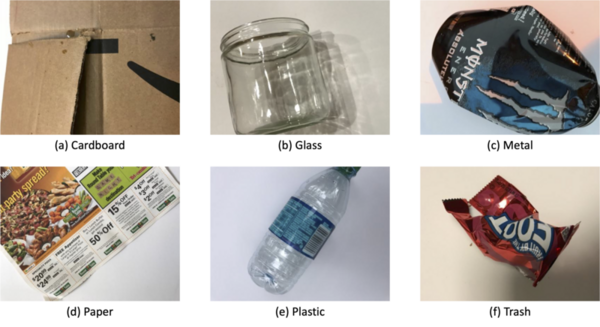
Classifying waste is a simple but useful exercise to protect the environment. Based on the success of deep learning, recent works have attempted to develop a waste classification model using deep neural networks. As we attempted to make our very own model to address waste classification, we recognized a common issue that other researchers reported as well: the lack of training data. This is an especially big problem when attempting to train a model for a task that has not been commonly explored before. Further research led us to federated learning (FL) for a solution, as it allows participants to aid in training the model using their own data. However, instead of sending the data from the clients to the server for training, FL trains the model on the clients’ machines using the clients’ data, and then aggregates the trained models into the server’s model to protect the clients’ private information. This means that there is a higher chance that the data used to train local models are heterogeneous, decreasing the overall performance. To overcome this issue, we ran a multitude of tests to determine how to maximize performance. For these tests, we hypothesized that the impact of data heterogeneity on an FL framework can be diminished through increasing the client participation ratio. Then, we measured the accuracies of the models trained with varying data heterogeneity, participation ratio, and the number of clients. From the results, we discovered that with less clients, having a higher participation ratio resulted in less accuracy degradation by the data heterogeneity.
This article has been tagged with: