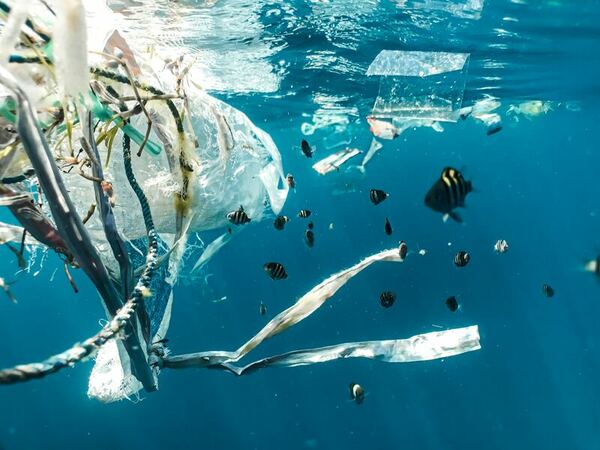
Here recognizing the growing amount of plastic waste in the oceans, the authors sought to develop and test laser imaging for the identification of waste in water. They found that while possible, limitations such as increasing depth and water turbidity result in increasing blurriness in laser images. While their image processing methods were somewhat insufficient they identified recent methods to use deep learning-based techniques as a potential avenue to viability for this method.
Read More...