Vineyard vigilance: Harnessing deep learning for grapevine disease detection
(1) Lexington High School, (2) Department of Biological Engineering, MIT
https://doi.org/10.59720/23-251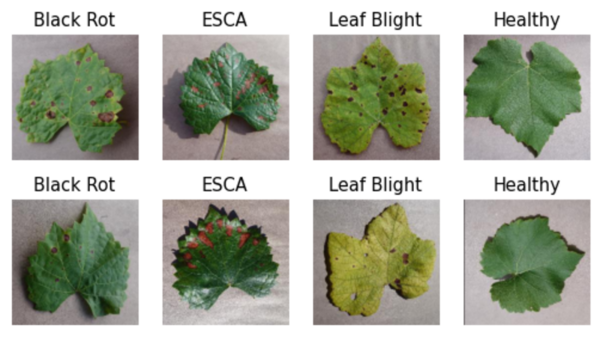
According to the International Organization of Vine and Wine, an impressive 77.8 million tons of grapes are cultivated globally each year. Grapes, long a cornerstone of both diets and agriculture worldwide, are increasingly threatened by diseases such as black rot, Esca, and leaf blight. In modern grapevine orchards, the prevalent method of disease detection relies on human observation, a process that often leads to delays in identifying these afflictions. This inefficiency typically results in diminished crop yields and inferior fruit quality. Automating grapevine leaf disease detection using machine learning will lead to sustainable farming. Deep learning, a branch of machine learning, is well-suited for learning from image data. In this paper, we deployed Convolutional Neural Networks (CNN), a type of deep learning model, to accurately classify healthy and disease-affected grapevine leaf images. We developed a baseline CNN model along with several transfer learning CNN models, namely DenseNet121, EfficientNetB7, MobileNetV2, ResNet50, and VGG16. We hypothesized that ResNet50 would be the best-performing model as it allows deep networks to train with high accuracy, but EfficientNetB7 turned out to be the most accurate model after experimentation. We created a max-voting ensemble with the three most accurate CNN models from the set, and it delivered better results than any individual CNN model. Our max-voting ensemble was then deployed on the web. Consequently, grapevine farmers and other users can detect the three common grapevine leaf diseases – black rot, Esca, and leaf blight, as well as healthy leaves by uploading their own images from the orchard.
This article has been tagged with: