Propagation of representation bias in machine learning
(1) Westford Academy, Westford, MA
https://doi.org/10.59720/20-113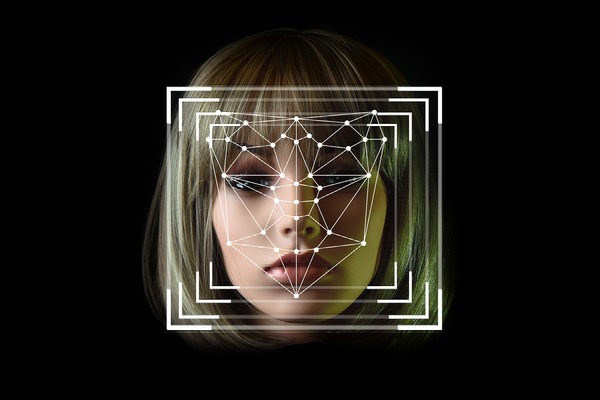
Transfer learning is an emerging paradigm in machine learning that involves reusing existing pretrained models to develop new machine learning applications. As machine learning grows in importance, keeping new models and applications clear of biases is of paramount importance. While previous works focus on the development of bias free models, they fail to address the mechanism of bias propagation in transfer learning. Using facial recognition as a use-case scenario, we attempt to identify sources of bias in a model developed using transfer learning. To achieve this task, we developed a model based on a pre-trained facial recognition model, and scrutinized the accuracy of the model’s image classification against factors such as age, gender, and race to observe whether or not the model performed better on some demographic groups than others. By identifying the bias and finding potential sources of bias, his work contributes a unique technical perspective from the view of a small scale developer to emerging discussions of accountability and transparency in AI.
This article has been tagged with: