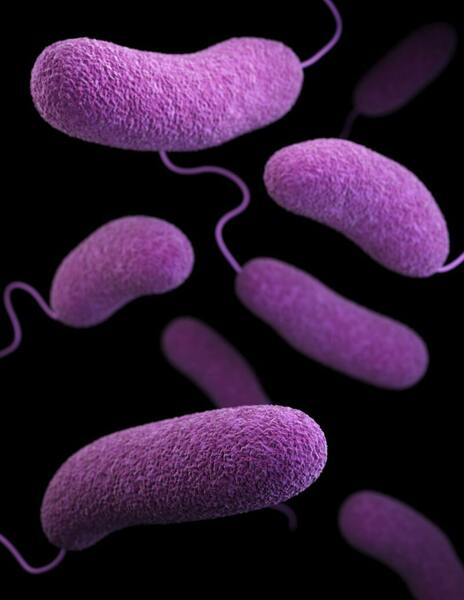
In this study, the authors test new antimicrobials by measuring the ability of extracts from Australian-native Myrtaceae species to induce death of two bacteria S. aureus and P. aeruginosa.
Read More...Myrtaceaes as antimicrobial agents against Staphylococcus aureus and Pseudomonas aeruginosa
In this study, the authors test new antimicrobials by measuring the ability of extracts from Australian-native Myrtaceae species to induce death of two bacteria S. aureus and P. aeruginosa.
Read More...Exercise, grades, stress, and learning experiences during remote learning due to the COVID-19 pandemic
In this study, the authors survey middle and high school students in different states in the U.S. to evaluate stress levels, learning experiences, and activity levels during the COVID-19 pandemic.
Read More...Wound healing properties of mesenchymal conditioned media: Analysis of PDGF, VEGF and IL-8 concentrations
Regenerative medicine has become a mainstay in recent times, and employing stem cells to treat several degenerative, inflammatory conditions has resulted in very promising outcomes. These forms of cell-based therapies are novel approaches to existing treatment modalities. In this study, the authors compared the concentrations of the cytokines PDGF, IL-8, and VEGF between conditioned and spent media of mesenchymal stem cells (MSCs) to evaluate their potential therapeutic properties for wound healing in inflammatory conditions. They hypothesized that conditioned media contains higher concentrations of wound healing cytokines compared to spent media. The authors found that while IL-8 and VEGF were present in highest concentrations in conditioned media, PDGF was present in maximal amounts in spent media.
Read More...Formulation of novel polyherbal compound MAT20 with phytochemicals found in amla, tulsi, and moringa
With herbal plants providing an address to the adverse effects of oxidative stress found within the body, the authors of this article develop and assess a novel compound (“MAT20”) that blends three herbal plants for optimal oxidative stress relief.
Read More...Impact of study partner status and group membership on commitment device effectiveness among college students
Here seeking to identify a possible solution to procrastination among college students, the authors used an online experiment that involved the random assignment of study partners that they shared their study time goal with. These partners were classified by status and group membership. The authors found that status and group membership did not significantly affect the likelihood of college students achieving their committed goals, and also suggest the potential of soft commitment devices that take advantage of social relationships to reduce procrastination.
Read More...Developing “Off the Shelf” Pancreases for Diabetic Patients Using Bacterial and Kombucha Tea Waste
In this study, the authors investigate the suitability of using bacterial cellulose as a scaffold for cell transplants. Interestingly, this cellulose is a can be found in the discard from a symbiotic culture of bacteria and yeast (SCOBY) used to make kombucha.
Read More...Synthetic auxin’s effect on root hair growth and peroxisomes in Arabidopsis thaliana
The authors looked at the ability of synthetic auxin to increase root hair growth in Arabidopsis thaliana. They found that 0.1 µM synthetic auxin significantly increased root hair length, but that 0.01 µM and 1 µM did not have any significant effect.
Read More...Computational analysis and drug repositioning: Targeting the TDP-43 RRM using FDA-approved drugs
Molecules which bind to proteins that aggregate abnormally in neurodegenerative diseases could be promising drugs for these diseases. In this study, Zhang, Wu, Zhang, and Dang simulate the binding behavior of various molecules to screen for candidates which could be promising candidates for drug development.
Read More...Using explainable artificial intelligence to identify patient-specific breast cancer subtypes
Breast cancer is the most common cancer in women, with approximately 300,000 diagnosed with breast cancer in 2023. It ranks second in cancer-related deaths for women, after lung cancer with nearly 50,000 deaths. Scientists have identified important genetic mutations in genes like BRCA1 and BRCA2 that lead to the development of breast cancer, but previous studies were limited as they focused on specific populations. To overcome limitations, diverse populations and powerful statistical methods like genome-wide association studies and whole-genome sequencing are needed. Explainable artificial intelligence (XAI) can be used in oncology and breast cancer research to overcome these limitations of specificity as it can analyze datasets of diagnosed patients by providing interpretable explanations for identified patterns and predictions. This project aims to achieve technological and medicinal goals by using advanced algorithms to identify breast cancer subtypes for faster diagnoses. Multiple methods were utilized to develop an efficient algorithm. We hypothesized that an XAI approach would be best as it can assign scores to genes, specifically with a 90% success rate. To test that, we ran multiple trials utilizing XAI methods through the identification of class-specific and patient-specific key genes. We found that the study demonstrated a pipeline that combines multiple XAI techniques to identify potential biomarker genes for breast cancer with a 95% success rate.
Read More...Structure-activity relationship of berberine and G4 DNA reveals aromaticity’s effect on binding affinity
Berberine is a natural quaternary alkaloid that has anti-microbial and anti-cancer effects. This compound can bind to Guanine Quadruplex (G4) DNA secondary complexes to help inhibit cancer cell proliferation. In this study, the authors investigate whether incorporating large aromatic rings helps to stabilize berberine-G4 interactions.
Read More...Search articles by title, author name, or tags