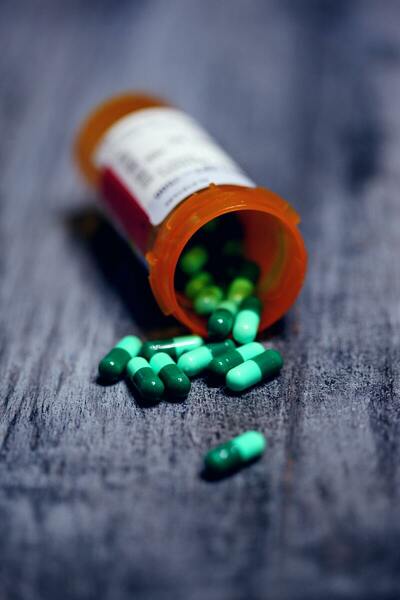
The authors investigate how improper disposal of medication can be mitigated through community education efforts.
Read More...Survey of medication disposal: Patient views and awareness
The authors investigate how improper disposal of medication can be mitigated through community education efforts.
Read More...Friend or foe: Using DNA barcoding to identify arthropods found at home
Here the authors used morphological characters and DNA barcoding to identify arthropods found within a residential house. With this method they identified their species and compared them against pests lists provided by the US government. They found that none of their identified species were considered to be pests providing evidence against the misconception that arthropods found at home are harmful to humans. They suggest that these methods could be used at larger scales to better understand and aid in mapping ecosystems.
Read More...Attitudes towards mental health in Indians who practice yoga regularly and those who do not
Whether it is through implicit association or intentional practice, yoga has been known to help individuals maintain good mental health. However, many communities, such as South Asian communities, often project the stereotype that embodies neglecting topics such as mental health and considering them taboo. In this online survey-based study, the authors focused on examining whether yoga would alter individuals’ attitudes toward mental health. They hypothesized that 1) participants who regularly practiced yoga would be more familiar with the term mental health, 2) participants who practiced yoga would value their mental health more, and 3) participants who practiced yoga regularly would be more open about their mental health and be more likely to reach out for professional help if needed. They did not find a statistical significance for any of our hypotheses which suggests that yoga may not have an effect on perceptions of mental health in yoga-practicing Indian adults.
Read More...Assigning Lightning Seasons to Different Regions in the United States
Climate change is predicted to increase the frequency of severe thunderstorm events in coming years. In this study, the authors hypothesized that (i) the majority of severe thunderstorm events will occur in the summer months in all states examined for all years analyzed, (ii) climate change will cause an unusual number of severe thunderstorm events in winter months in all states, (iii) thundersnow would be observed in Colorado, and (iv.) there would be no difference in the number of severe thunderstorm events between states in any given year examined. They classified lightning seasons in all states observed, with the most severe thunderstorm events occurring in May, June, July, and August. Colorado, New Jersey, Washington, and West Virginia were found to have severe thunderstorm events in the winter, which could be explained by increased winter storms due to climate change (1). Overall, they highlight the importance of quantifying when lightning seasons occur to avoid lightning-related injuries or death.
Read More...Studying habitability of the exoplanents Kepler-504 b, Kepler-315 b, and Kepler-315 c
The authors explore how similar exoplanets are to Earth and whether they could be inhabited by humans and other living organisms.
Read More...Ramifications of natural and artificial sweeteners on the gastrointestinal system
This study aimed to determine whether artificial sweeteners are harmful to the human microbiome by investigating two different bacteria found to be advantageous to the human gut, Escherichia coli and Bacillus coagulans. Results showed dramatic reduction in bacterial growth for agar plates containing two artificial sweeteners in comparison to two natural sweeteners. This led to the conclusion that both artificial sweeteners inhibit the growth of the two bacteria and warrants further study to determine if zero-sugar sweeteners may be worse for the human gut than natural sugar itself.
Read More...Deep residual neural networks for increasing the resolution of CCTV images
In this study, the authors hypothesized that closed-circuit television images could be stored with improved resolution by using enhanced deep residual (EDSR) networks.
Read More...A study of South Korean international school students: Impact of COVID-19 on anxiety and learning habits
In this study, the authors investigate the effects of the COVID-19 pandemic on South Korean international school students' anxiety, well being and their learning habits.
Read More...Recognition of animal body parts via supervised learning
The application of machine learning techniques has facilitated the automatic annotation of behavior in video sequences, offering a promising approach for ethological studies by reducing the manual effort required for annotating each video frame. Nevertheless, before solely relying on machine-generated annotations, it is essential to evaluate the accuracy of these annotations to ensure their reliability and applicability. While it is conventionally accepted that there cannot be a perfect annotation, the degree of error associated with machine-generated annotations should be commensurate with the error between different human annotators. We hypothesized that machine learning supervised with adequate human annotations would be able to accurately predict body parts from video sequences. Here, we conducted a comparative analysis of the quality of annotations generated by humans and machines for the body parts of sheep during treadmill walking. For human annotation, two annotators manually labeled six body parts of sheep in 300 frames. To generate machine annotations, we employed the state-of-the-art pose-estimating library, DeepLabCut, which was trained using the frames annotated by human annotators. As expected, the human annotations demonstrated high consistency between annotators. Notably, the machine learning algorithm also generated accurate predictions, with errors comparable to those between humans. We also observed that abnormal annotations with a high error could be revised by introducing Kalman Filtering, which interpolates the trajectory of body parts over the time series, enhancing robustness. Our results suggest that conventional transfer learning methods can generate behavior annotations as accurate as those made by humans, presenting great potential for further research.
Read More...Using machine learning to develop a global coral bleaching predictor
Coral bleaching is a fatal process that reduces coral diversity, leads to habitat loss for marine organisms, and is a symptom of climate change. This process occurs when corals expel their symbiotic dinoflagellates, algae that photosynthesize within coral tissue providing corals with glucose. Restoration efforts have attempted to repair damaged reefs; however, there are over 360,000 square miles of coral reefs worldwide, making it challenging to target conservation efforts. Thus, predicting the likelihood of bleaching in a certain region would make it easier to allocate resources for conservation efforts. We developed a machine learning model to predict global locations at risk for coral bleaching. Data obtained from the Biological and Chemical Oceanography Data Management Office consisted of various coral bleaching events and the parameters under which the bleaching occurred. Sea surface temperature, sea surface temperature anomalies, longitude, latitude, and coral depth below the surface were the features found to be most correlated to coral bleaching. Thirty-nine machine learning models were tested to determine which one most accurately used the parameters of interest to predict the percentage of corals that would be bleached. A random forest regressor model with an R-squared value of 0.25 and a root mean squared error value of 7.91 was determined to be the best model for predicting coral bleaching. In the end, the random model had a 96% accuracy in predicting the percentage of corals that would be bleached. This prediction system can make it easier for researchers and conservationists to identify coral bleaching hotspots and properly allocate resources to prevent or mitigate bleaching events.
Read More...Search articles by title, author name, or tags