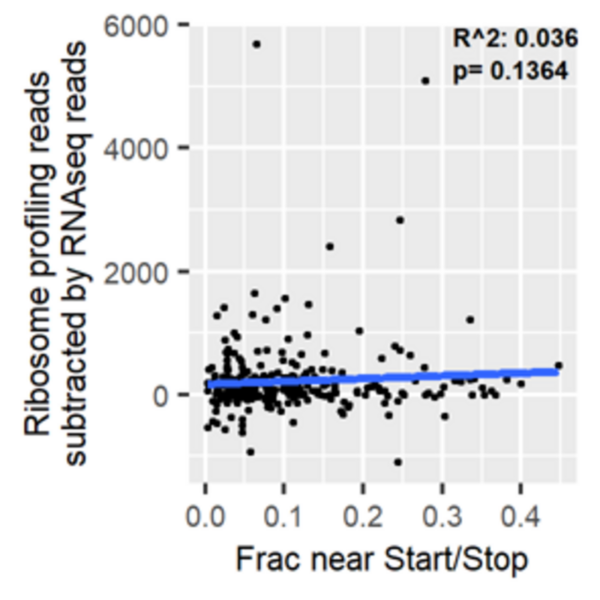
In this article, the authors analyzed ribosome profiling data from amino acid-starved pancreatic cancer cells to explore whether the pattern of ribosome distribution along transcripts under normal conditions can predict the degree of ribosome stalling under stress. The authors found that ribosomes in amino acid-deprived cells stalled more along elongation-limited transcripts. By contrast, they observed no relationship between read density near start and stop and disparities between mRNA sequencing reads and ribosome profiling reads. This research identifies an important relationship between read distribution and propensity for ribosomes to stall, although more work is needed to fully understand the patterns of ribosome distribution along transcripts in ribosome profiling data.
Read More...