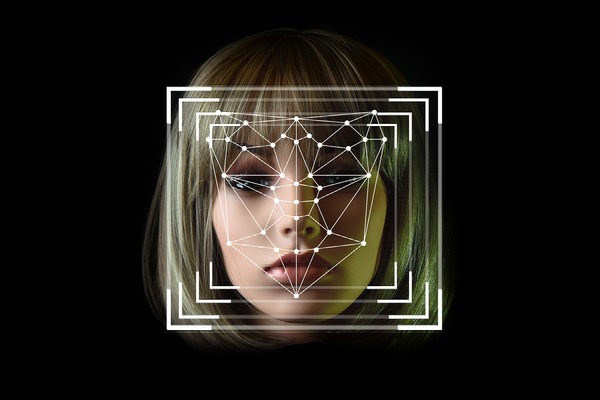
Using facial recognition as a use-case scenario, we attempt to identify sources of bias in a model developed using transfer learning. To achieve this task, we developed a model based on a pre-trained facial recognition model, and scrutinized the accuracy of the model’s image classification against factors such as age, gender, and race to observe whether or not the model performed better on some demographic groups than others. By identifying the bias and finding potential sources of bias, his work contributes a unique technical perspective from the view of a small scale developer to emerging discussions of accountability and transparency in AI.
Read More...