Battling cultural bias within hate speech detection: An experimental correlation analysis
(1) Chagrin Falls High School
https://doi.org/10.59720/23-156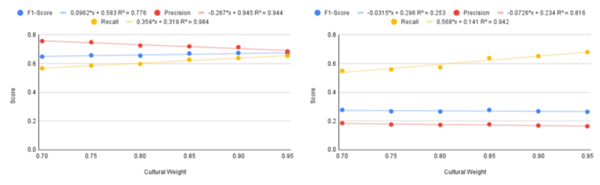
Hate speech detection systems have become essential in the advancing digital world. They limit the dissemination of hateful and offensive language online. However, the machine learning algorithms that provide the basis for these systems struggle to identify hate speech versus clean speech within a cultural context, allowing the growth of cultural bias. Though previous methods had aimed to mitigate the cultural bias of a machine learning model, we attempted to find a new understanding with regard to cultural bias. This study sought to determine a correlation between increasing the amount of cultural speech used to train the machine learning model and the model’s cultural bias when classifying hate speech and clean speech. Additionally, we hypothesized that increasing the cultural weight of a training dataset would mitigate the cultural bias. To test this hypothesis, we created a unique method named Categorial Weighted Training (CaWT), derived from multiple other methods of previous researchers, to identify a correlation. CaWT involved the creation of multiple culturally-weighted training datasets and training a machine learning algorithm against them. From this, the results illustrated minimal correlation between the cultural weight of a training dataset and the model’s performance on cultural speech. However, a significant negative correlation exists between the cultural weight and the model’s performance on non-cultural speech. This implies that increasing the cultural weight does not affect the model’s cultural bias but decreases the model’s performance on non-cultural speech, suggesting that a lower cultural weight is ideal within the limitations of our research.
This article has been tagged with: