A HOG feature extraction and CNN approach to Parkinson’s spiral drawing diagnosis
(1) Edison Academy Magnet School, (2) Systems Engineering & Integration, Illumina
https://doi.org/10.59720/24-048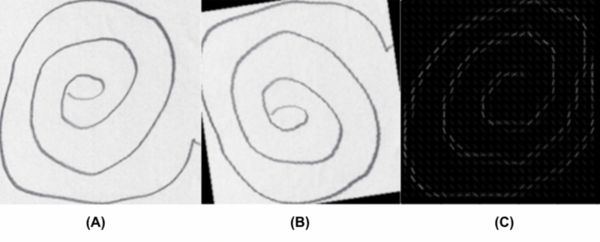
Parkinson’s disease (PD) is the second most widespread neurodegenerative disorder in the United States following Alzheimer’s disease. Its inefficient and often inaccessible diagnostic process relies heavily on a healthcare professional’s analysis of a patient’s performance on neurological and physical exams. We aim to use machine and deep learning to improve the efficiency, accessibility, and accuracy of the diagnosis process by utilizing the effect that rhythmic shaking in the hand, a common PD symptom, has on a patient's ability to draw a spiral. We used images of spirals drawn by both PD and non-PD individuals to train a deep learning model that relied strictly on computer vision and a support vector machine (SVM)-based machine learning model that utilized histogram of oriented gradients feature extraction. We hypothesized that both models would outperform the current clinical diagnosis process by reducing misdiagnosis rates. This hypothesis was unsupported by our study’s findings. The models are unable to distinguish between PD and other neurocognitive disorders like multiple sclerosis or essential tremor. The models, therefore, cannot be directly compared to the current clinical diagnosis process. Instead, both models, in general, can be used as an efficient and highly accessible baseline diagnosis tool for neurocognitive disorders, complementing, rather than replacing, clinical diagnosis methods to improve the accessibility and efficiency of an accurate neurocognitive diagnosis.
This article has been tagged with: