A novel approach for predicting Alzheimer’s disease using machine learning on DNA methylation in blood
(1) San Dieguito High School Academy, Encinitas, California, (2) Stanford University, Stanford, California
https://doi.org/10.59720/23-054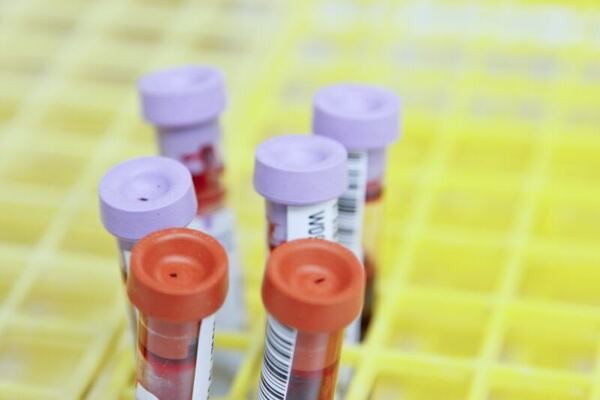
Alzheimer’s disease (AD) is the leading cause of dementia worldwide. Mild cognitive impairment (MCI) is an early stage of mental decline that can precede the development of AD. Oftentimes, the progression of MCI to AD is difficult to predict. An AD diagnosis can involve invasive brain scans and spinal fluid tests. However, one promising biomarker for the diagnosis of AD is epigenetic data, specifically the methylation level of cytosine-phosphate-guanine (CpG) sites in the DNA. AD is linked to environmental factors, with some factors causing changes in these DNA methylation levels. We hypothesized that machine learning models can use blood DNA methylation levels, sex, and age to predict between cognitive normality, MCI, and AD with at least 50% accuracy. In this paper, we generated four machine learning models and two dataset dimensionality reduction methods in order to test this hypothesis. We trained the models on data from CpG sites from whole blood. When predicting if a patient would be cognitively normal, have MCI, or have AD, we achieved an accuracy of 53.33%, which is 20% greater than random guessing. We achieved this accuracy using a gradient boosting decision trees model in combination with a logistic regression method for feature selection. While this accuracy is low, the feature selection method that we developed may be useful in future research, as it identifies CpG sites that are most correlated with AD. Since peripheral blood is easily accessible through blood draw, our model represents a practical way to assist in the diagnosis of AD.
This article has been tagged with: