Utilizing meteorological data and machine learning to predict and reduce the spread of California wildfires
(1) Aspiring Scholars Directed Research Program
* These authors made equal contributions
https://doi.org/10.59720/22-163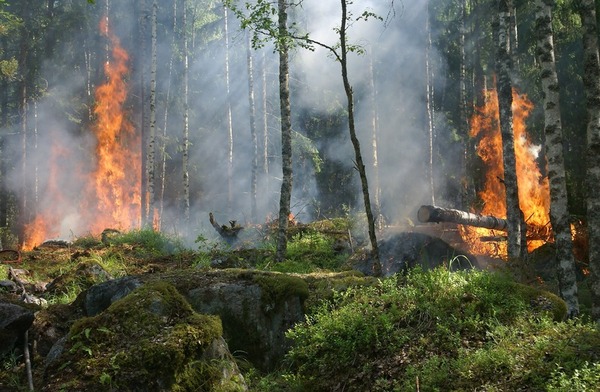
With the increase in the number and severity of California wildfires, firefighters need more efficient tools to predict potential wildfires so that they can efficiently cease these devastating events, preserve the safety of the human population, and the environment. With nearly eight million acres burned between 2010 and 2019, there is a clear and immediate issue. We hypothesized that a machine learning model could be developed to accurately predict the severity of California wildfires and determine the most influential meteorological factors. In this study, we used machine learning to analyze historical fire behaviors and then accurately predict the severity (defined by acres burned) of California wildfires. We created a custom dataset by using a combination of information from the World Weather Online API and a Kaggle dataset of wildfires in California from 20132020. The resulting dataset consisted of information (including temperature, humidity, dew point, and wind speeds) on 1,462 wildfires. We used three classification algorithms—logistic regression, support vector machine, and random forest regression—to classify these fires. Devising a system categorizing fires based on acres burned, our algorithms were able to classify fires into seven categories with promising accuracy (around 55 percent). We generated a correlation matrix, providing insight into the most concerning factors and allowing firefighters to choose the best course of action. We found that higher temperatures, lower humidity, lower dew point, higher wind gusts, and higher wind speeds are the most significant contributors to the spread of a wildfire. This machine learning tool could vastly improve the efficiency and preparedness of firefighters as they deal with these crises.
This article has been tagged with: