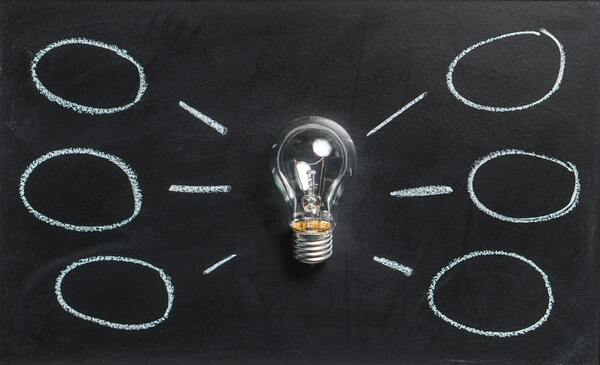
The purpose of the study was to determine whether graph-based machine learning techniques, which have increased prevalence in the last few years, can accurately classify data into one of many clusters, while requiring less labeled training data and parameter tuning as opposed to traditional machine learning algorithms. The results determined that the accuracy of graph-based and traditional classification algorithms depends directly upon the number of features of each dataset, the number of classes in each dataset, and the amount of labeled training data used.
Read More...