Quantitative analysis and development of alopecia areata classification frameworks
(1) Meridian World School
https://doi.org/10.59720/24-004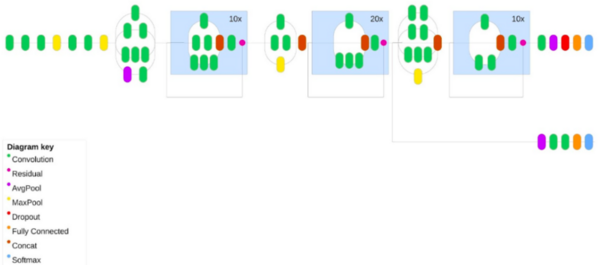
Alopecia areata is an autoimmune disorder resulting in rapid and unpredictable hair loss on the scalp or body as the immune system mistakenly attacks human hair follicles. In the United States alone, about 6.7 million people experience a form of Alopecia. Early identification of the condition has shown notable potential in improving treatment outcomes and reducing complications. To diagnose Alopecia, researchers have proposed the use of deep learning (DL) techniques to classify images of hair as healthy or alopecia-affected, which has shown high potential. However, the research implementing relevant DL algorithms in the field of hair loss detection and estimation is limited. This paper presents a comparative analysis of our two newly optimized Convolutional neural networks (CNN) with other existing models. For training, we considered datasets comprised of images of healthy hair and alopecia-affected hair. Due to data unavailability, we gathered images of alopecia-affected hair from two distinct datasets: one from Figaro1k and one independently created dataset. After training the algorithms, we performed a contrastive assessment to determine the most effective one based on relevant criteria. We hypothesized that the initial performance of the base neural network would be closely connected to the subsequent accuracy of the algorithm when training it for a new task. As expected, the modified Inception-Resnet-v2 model achieved the greatest performance, with a validation accuracy and loss of 97.94% and 10.4%, respectively. The experimental results indicated that the proposed algorithm serves as an effective framework for Alopecia Areata classification.
This article has been tagged with: