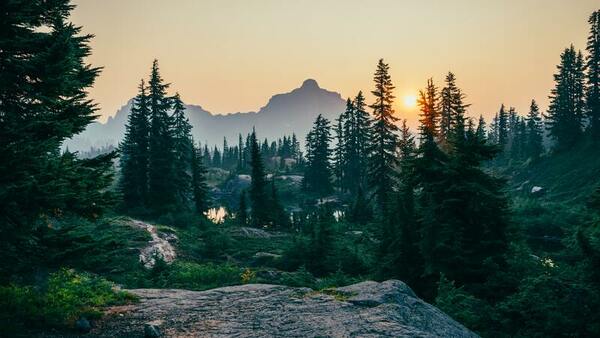
Here, seeking to identify an optimal method to classify tree species through remote sensing, the authors used a few machine learning algorithms to classify forest tree species through multispectral satellite imagery. They found the Random Forest algorithm to most accurately classify tree species, with the potential to improve model training and inference based on the inclusion of other tree properties.
Read More...