Risk assessment modeling for childhood stunting using automated machine learning and demographic analysis
(1) Conestoga High School, Berwyn, Pennsylvania, (2) Department of Biostatistics, Epidemiology and Informatics, Perelman School of Medicine, University of Pennsylvania, Philadelphia, Pennsylvania; Department of Computational Biomedicine, Cedars-Sinai Medical Center, Los Angeles, California
https://doi.org/10.59720/22-073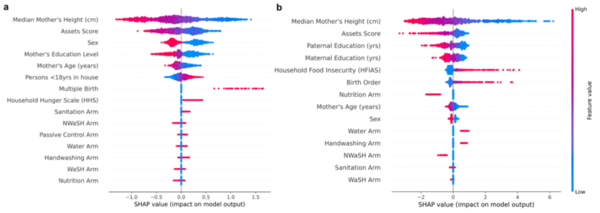
Over the last few decades, childhood stunting has persisted as a major global challenge. More than 154 million children under five are stunted across the world, with 95% of them residing in Asia and Africa alone. Apart from the small stature, stunting poses additional impediments including delayed cognitive development and health complications. Furthermore, the involvement of several demographic factors and limited timeframe of intervention effectiveness poses challenges to existing treatments. Thus, understanding the impact of demographic conditions on stunting and developing predictive methods is vital for advancing future research developing predictive models. In this study, we hypothesized that TPTO (Tree-based Pipeline Optimization Tool), an AutoML (automated machine learning) tool, would outperform all pre-existing machine learning models and reveal the positive impact of economic prosperity, strong familial traits, and resource attainability on reducing stunting risk. The TPOT pipelines, for data sets from Kenya and Bangladesh, outscored previously reported ML models with most performance metrics improving by 0.1 or more. Feature correlation plots revealed that maternal height, wealth indicators, and parental education were universally important features for determining stunting outcomes approximately two years after birth. Additionally, the machine learning models trained by TPOT resulted in strong overall performances with the best metrics scoring over 0.8. These results help inform future research by highlighting how demographic, familial, and socio-economic conditions influence stunting and providing medical professionals with a deployable risk assessment tool for predicting childhood stunting.
This article has been tagged with: