Groundwater prediction using artificial intelligence: Case study for Texas aquifers
(1) Awty International School, (2) London Stock Exchange Group (LSEG)
https://doi.org/10.59720/23-200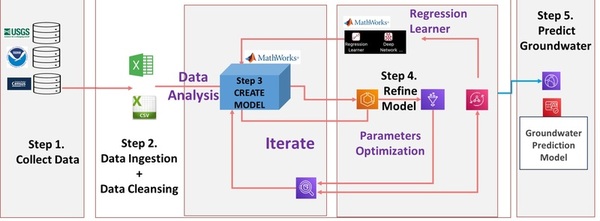
Groundwater resources are highly dependent upon recharge from precipitation; hence, variability in precipitation patterns is important for sustainable groundwater management. Climate change is not only increasing the frequency and intensity of extreme hydro-meteorological events like hurricanes and cyclones, but is also having an even more complex impact on other variables such as precipitation, which can be measured by the Palmer Modified Drought Index (PMDI). Therefore, it is imperative to understand how climate change impacts groundwater management. This research has created a preliminary model to predict the future groundwater levels based on the critical causality and regression analysis using artificial intelligence. In this study, we hypothesized that tree-based automated artificial intelligence models, would perform best in predicting future groundwater levels. Unlike other machine learning models, tree-based models can accommodate correlated datasets like climate variables and changing demographics. We acquired water aquifer data from the United States Geological Survey (USGS) for Texas aquifers, climate data from the National Oceanic and Atmospheric Administration (NOAA), and population data from the US census to train artificial intelligence models using MATLAB. This research project identified key trends that groundwater levels were down 50% on a normalized basis for the selected dataset and established correlation of groundwater changes, weather related changes (temperature changes, precipitation changes and drought indices) and demographic changes. Understanding these key trends allowed us to select the right predictors for the AI model. Tree-based AI models predicted the future groundwater levels with the most accuracy and least root mean square error (RMSE) as compared to other AI models like linear regression, neural network, support vector machines.
This article has been tagged with: