Prediction of diabetes using supervised classification
(1) American High School, (2) Horizon Academic Research Program
https://doi.org/10.59720/23-062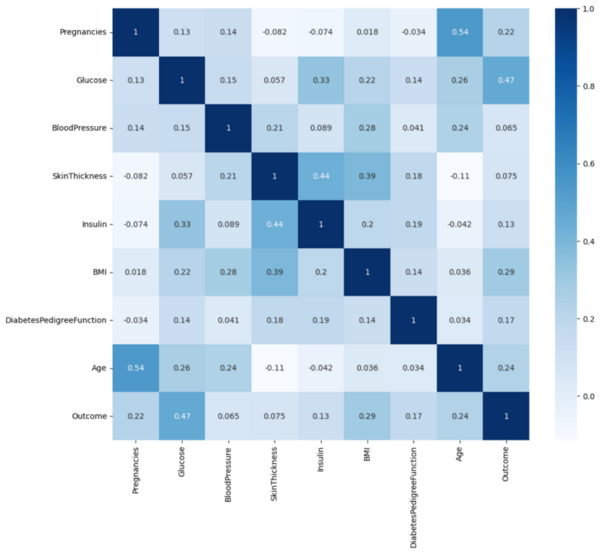
Diabetes is one of the common chronic diseases that impacts 28.7 million people in the US as of 2019, accounting for 8.7% of the total population. Early identification of diabetes is very important in disease control and management. A number of prior studies provided compelling evidence that machine learning can help identify diabetes early allowing for timely treatment. It remains a challenge to appropriately assess, optimize and refine the classification models based on specific dataset for diabetes prediction with high accuracy. In this study, we aimed to develop a model with improved accuracy for diabetes prediction. We employed six learning algorithms, logistic regression, k-nearest neighbors (k-NN), support vector machine (SVM), decision tree, random forest, and gradient boosting on the Pima Indians Diabetes Dataset. The performance of each model was evaluated for the prediction of diabetes in validation datasets using accuracy, precision, recall, and F1-score. Gradient boosting provided an accuracy of 81.8%, outperforming all other classification models in most of the performance measures. Collectively, the gradient boosting model appeared to provide an appropriate algorithm for diabetes prediction with high accuracy based on the diagnostic measurements gathered in this specific dataset. Of note, the insights yielded from this exploratory study may only be applicable to this subpopulation of diabetes patients. It remains to be further validated with datasets derived from more diverse diabetes populations before the findings can be generalized to a wider diabetes patient population.
This article has been tagged with: