Predicting asthma-related emergency department visits and hospitalizations with machine learning techniques
(1) Saint Francis High School, Mountain View, California, (2) Schmahl Science Workshops, San Jose, California
https://doi.org/10.59720/21-074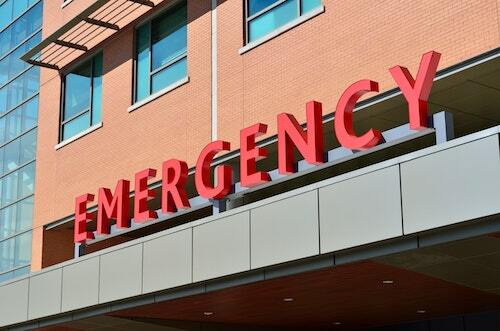
With the World Health Organization’s declaration that 90% of the world breathes hazardous air, there is an increasing need to investigate the effects of ambient pollutants on human respiratory health. This research uses machine learning (ML) to examine asthma in Los Angeles County, an area with substantial pollution, and determine the success of classifiers in predicting future asthma-related hospitalizations. The objectives of applying ML-based solutions into healthcare are trifold. Firstly, it identifies the ML models that most accurately predict asthma hospitalizations. Secondly, it evaluates the significance of the correlation among ambient pollution, weather, and asthma. Ultimately, the model serves as a clinical support system to forewarn health care providers of asthma exacerbations. The hypothesis was that ML classification techniques would be able to predict asthmatic census based on ambient pollution and weather, displaying a positive trend between pollutant levels and asthma hospitalizations. The models revealed that nitrogen dioxide (NO2) and ozone (O3) levels were significantly correlated with asthma hospitalizations. A simple decision tree was the least accurate but useful in selecting features for other models. K-nearest neighbors, random forest, gradient boosting, and support vector machine classifiers predicted the asthma quartile with accuracies of 68%, 65%, 64%, and 62%, respectively. Overall, four ML classifiers were promising predictors, all showing consistency in k-fold crossover testing. The seasonal surge in asthma hospitalizations suggests that further research should explore other seasonal variables and ML classifiers to improve the models.
This article has been tagged with: