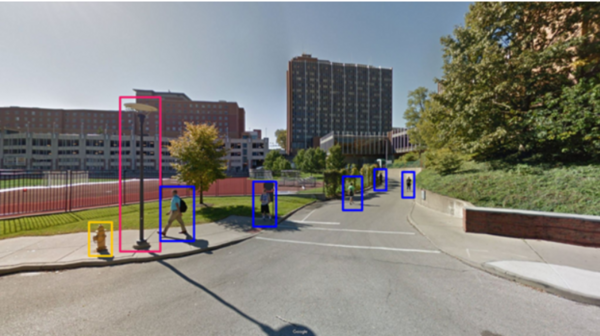
Every year, around 40% of undergraduate students in the United States discontinue their studies, resulting in a loss of valuable education for students and a loss of money for colleges. Even so, colleges across the nation struggle to discover the underlying causes of these high dropout rates. In this paper, the authors discuss the use of machine learning to find correlations between the built environment factors and the retention rates of colleges. They hypothesized that one way for colleges to improve their retention rates could be to improve the physical characteristics of their campus to be more pleasing. The authors used image classification techniques to look at images of colleges and correlate certain features like colors, cars, and people to higher or lower retention rates. With three possible options of high, medium, and low retention rates, the probability that their models reached the right conclusion if they simply chose randomly was 33%. After finding that this 33%, or 0.33 mark, always fell outside of the 99% confidence intervals built around their models’ accuracies, the authors concluded that their machine learning techniques can be used to find correlations between certain environmental factors and retention rates.
Read More...