Monitoring drought using explainable statistical machine learning models
(1) Notre Dame San Jose High School, (2) AIClub
https://doi.org/10.59720/23-026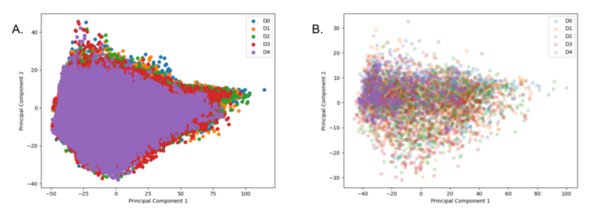
Droughts have a wide range of effects, from ecosystems failing and crops dying, to increased illness and decreased water quality. Drought prediction is important because it can help communities, businesses, and governments plan and prepare for these detrimental effects. This study predicts drought conditions by using predictable weather patterns in machine learning models. The model utilized factors such as wind speed, temperature, and humidity to better understand the importance of certain features in drought prediction. We hypothesized that explainable machine learning models can effectively forecast future drought severity. Results of experiments with a public domain data set showed that explainable statistical machine learning models predict drought severity with average precision and recall values of 0.55 and 0.56, respectively. We identified a subset of meteorological indicators as major contributors to predicting the occurrence of drought. The experiments used K- nearest neighbors (KNN) and random forest algorithms to predict drought severity. The random forest algorithm achieved the highest accuracy at 200 trees and 30 depth, while KNN achieved the highest accuracy when K was set to one. Feature importance analysis showed that surface pressure, temperature range at two meters, and maximum temperature at two meters were the most important features. In conclusion, statistical machine learning models provide insights into factors that impact drought and can enable researchers to identify steps to prevent natural disasters.
This article has been tagged with: