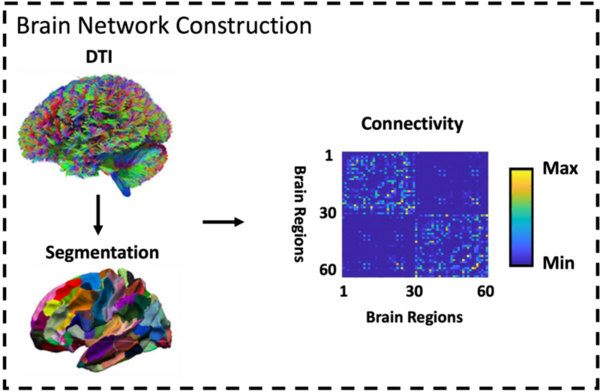
The authors compare neuroimaging datasets to identify potential new biomarkers for earlier detection of Alzheimer's disease.
Read More...Predictions of neural control deficits in elders with subjective memory complaints and Alzheimer’s disease
The authors compare neuroimaging datasets to identify potential new biomarkers for earlier detection of Alzheimer's disease.
Read More...Applying centrality analysis on a protein interaction network to predict colorectal cancer driver genes
In this article the authors created an interaction map of proteins involved in colorectal cancer to look for driver vs. non-driver genes. That is they wanted to see if they could determine what genes are more likely to drive the development and progression in colorectal cancer and which are present in altered states but not necessarily driving disease progression.
Read More...An explainable model for content moderation
The authors looked at the ability of machine learning algorithms to interpret language given their increasing use in moderating content on social media. Using an explainable model they were able to achieve 81% accuracy in detecting fake vs. real news based on language of posts alone.
Read More...Similarity Graph-Based Semi-supervised Methods for Multiclass Data Classification
The purpose of the study was to determine whether graph-based machine learning techniques, which have increased prevalence in the last few years, can accurately classify data into one of many clusters, while requiring less labeled training data and parameter tuning as opposed to traditional machine learning algorithms. The results determined that the accuracy of graph-based and traditional classification algorithms depends directly upon the number of features of each dataset, the number of classes in each dataset, and the amount of labeled training data used.
Read More...Building a video classifier to improve the accuracy of depth-aware frame interpolation
In this study, the authors share their work on improving the frame rate of videos to reduce data sent to users with both 2D and 3D footage. This work helps improve the experience for both types of footage!
Read More...Solving a new NP-Complete problem that resembles image pattern recognition using deep learning
In this study, the authors tested the ability and accuracy of a neural net to identify patterns in complex number matrices.
Read More...Changes in Aromanian language use and the Aromanian ethnolinguistic group’s reaction to decline
The Aromanian language and culture is quickly declining towards extinction. In this new research article, Ganea and Lascu provide evidence that, although the use of the Aromanian language is less prevalent among younger individuals, participants overwhelming support the preservation of Aromanian language and culture.
Read More...Developing a neural network to model the mechanical properties of 13-8 PH stainless steel alloy
We systematically evaluated the effects of raw material composition, heat treatment, and mechanical properties on 13-8PH stainless steel alloy. The results of the neural network models were in agreement with experimental results and aided in the evaluation of the effects of aging temperature on double shear strength. The data suggests that this model can be used to determine the appropriate 13-8PH alloy aging temperature needed to achieve the desired mechanical properties, eliminating the need for many costly trials and errors through re-heat treatments.
Read More...Pancreatic Adenocarcinoma: An Analysis of Drug Therapy Options through Interaction Maps and Graph Theory
Cancer is often caused by improper function of a few proteins, and sometimes it takes only a few proteins to malfunction to cause drastic changes in cells. Here the authors look at the genes that were mutated in patients with a type of pancreatic cancer to identify proteins that are important in causing cancer. They also determined which proteins currently lack effective treatment, and suggest that certain proteins (named KRAS, CDKN2A, and RBBP8) are the most important candidates for developing drugs to treat pancreatic cancer.
Read More...Using Artificial Intelligence to Forecast Continuous Glucose Monitor(CGM) readings for Type One Diabetes
People with Type One diabetes often rely on Continuous Blood Glucose Monitors (CGMs) to track their blood glucose and manage their condition. Researchers are now working to help people with Type One diabetes more easily monitor their health by developing models that will future blood glucose levels based on CGM readings. Jalla and Ghanta tackle this issue by exploring the use of AI models to forecast blood glucose levels with CGM data.
Read More...