An explainable model for content moderation
(1) Gretchen Whitney High School, Cerritos, California
https://doi.org/10.59720/22-288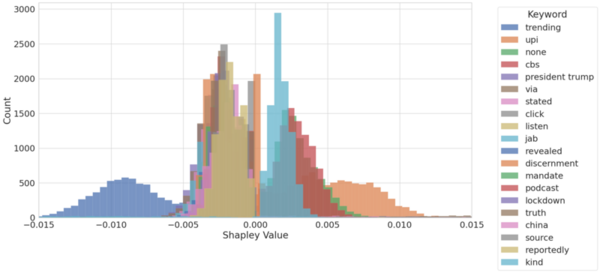
The spread of fake news on social media has eroded trust in traditional news outlets and institutions. In response, social media platforms have incorporated machine learning, algorithms that can learn patterns in data without explicit programming, into their content moderation systems to help remove fake posts. However, these algorithms often misinterpret language and make poor moderation decisions. We need to better understand how machine learning algorithms interpret language. Explainability is a challenge with neural networks, a popular machine-learning model inspired by how neurons communicate in the brain. In this study, we sought to develop an explainable model for content moderation comparable in accuracy to a traditional neural network, focusing on classifying real and fake news articles. We hypothesized that by identifying keywords and quantifying their contribution to an article’s credibility, we could predict credibility by summing the contributions of all the keywords in an article. We trained a convolutional neural network to classify articles using a 3,000-keyword vocabulary, achieving 85.8% accuracy. We used the Shapley additive explanations algorithm to calculate each keyword's median contribution to the model’s predictions. We created a linear model that summed the median contributions of keywords in an article, achieving a comparable 81.0% accuracy. We then examined keywords with the largest median contributions. Clickbait and COVID-19 terms correlated with fake news. Legal and political terminology correlated with real news. Our results demonstrate the potential for explainable models to improve our understanding of content moderation algorithms and fake news linguistics.
This article has been tagged with: