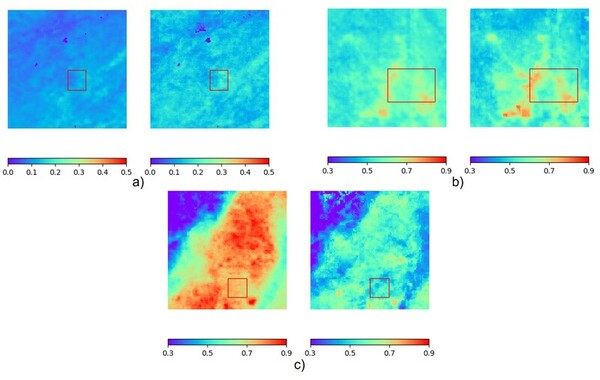
In this study, the authors use aerosol optical depth data to determine if aerosol levels were lower in major metropolitan areas around the world during the COVID-19 pandemic.
Read More...Analyzing aerosol variation during the COVID-19 pandemic lockdown using satellite data
In this study, the authors use aerosol optical depth data to determine if aerosol levels were lower in major metropolitan areas around the world during the COVID-19 pandemic.
Read More...Unlocking robotic potential through modern organ segmentation
The authors looked at different models of semantic segmentation to determine which may be best used in the future for segmentation of CT scans to help diagnose certain conditions.
Read More...Functional Network Connectivity: Possible Biomarker for Autism Spectrum Disorders (ASD)
Autism spectrum disorder (ASD) is a complex neurodevelopmental disorder and is difficult to diagnose in young children. Here magnetoencephalography was used to compare the brain activity in patients with ASD to patients in a control group. The results show that patients with ASD have a high level of activity in different areas of the brain than those in the control group.
Read More...Studying the effects of different anesthetics on quasi-periodic patterns in rat fMRI
The authors looked at the effects of commonly used anesthetics in rodents on brain activity (specifically quasi-periodic patterns). Understanding effects on brain activity is important for researchers to understand when choosing rodent models for disease.
Read More...Transfer Learning with Convolutional Neural Network-Based Models for Skin Cancer Classification
Skin cancer is a common and potentially deadly form of cancer. This study’s purpose was to develop an automated approach for early detection for skin cancer. We hypothesized that convolutional neural network-based models using transfer learning could accurately differentiate between benign and malignant moles using natural images of human skin.
Read More...A machine learning approach to detect renal calculi by studying the physical characteristics of urine
The authors trained a machine learning model to detect kidney stones based on characteristics of urine. This method would allow for detection of kidney stones prior to the onset of noticeable symptoms by the patient.
Read More...Starts and Stops of Rhythmic and Discrete Movements: Modulation in the Excitability of the Corticomotor Tract During Transition to a Different Type of Movement
Control of voluntary and involuntary movements is one of the most important aspects of human neurological function, but the mechanisms of motor control are not completely understood. In this study, the authors use transcranial magnetic stimulation (TMS) to stimulate a portion of the motor cortex while subjects performed either discrete (e.g. throwing) or rhythmic (e.g. walking) movements. By recording electrical activity in the muscles during this process, the authors showed that motor evoked potentials (MEPs) measured in the muscles during TMS stimulation are larger in amplitude for discrete movements than for rhythmic movements. Interestingly, they also found that MEPs during transitions between rhythmic and discrete movements were nearly identical and larger in amplitude than those recorded during either rhythmic or discrete movements. This research provides important insights into the mechanisms of neurological control of movement and will serve as the foundation for future studies to learn more about temporal variability in neural activity during different movement types.
Read More...Effect of Collagen Gel Structure on Fibroblast Phenotype
Environment affects the progression of life, especially at the cellular level. This study investigates multiple 3-dimensional growth environments, also known as scaffolds or hydrogels, and their effect on the growth of a type of cells called fibroblasts. These results suggest that a scaffold made of collagen and polyethylene glycol are favorable for cell growth. This research is useful for developing implantable devices to aid wound healing.
Read More...The use of computer vision to differentiate valley fever from lung cancer via CT scans of nodules
Pulmonary diseases like lung cancer and valley fever pose serious health challenges, making accurate and rapid diagnostics essential. This study developed a MATLAB-based software tool that uses computer vision techniques to differentiate between these diseases by analyzing features of lung nodules in CT scans, achieving higher precision than traditional methods.
Read More...Enhancing marine debris identification with convolutional neural networks
Plastic pollution in the ocean is a major global concern. Remotely Operated Vehicles (ROVs) have promise for removing debris from the ocean, but more research is needed to achieve full effectiveness of the ROV technology. Wahlig and Gonzales tackle this issue by developing a deep learning model to distinguish trash from the environment in ROV images.
Read More...