Unlocking robotic potential through modern organ segmentation
(1) Saratoga High School, (2) School of Clinical Medicine, University of Cambridge
https://doi.org/10.59720/23-323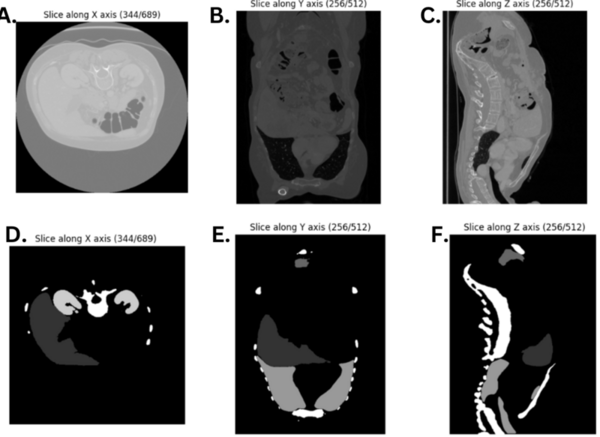
Deep learning has revolutionized the approach to complex, data-driven problems, specifically in medical imaging, where its techniques have significantly raised efficiency in organ segmentation. Enhancing the depth and precision of organ-based classification is an essential step towards automation of medical operations and diagnostics. Our study aimed to investigate the effect and potential advantages of different models using Binary Semantic Segmentation. We chose to employ the SegFormer model as our primary deep learning model because of its lightweight architecture, alongside different Unet variations. We hypothesized that the performance of the SegFormer model would surpass the different Convolutional Neural Networks (CNN) models. We assembled a custom 2D computerized tomography (CT) scan dataset CT-Org2D, through conversion from 3D volumes and placing them in their respective folders. In contrast to the selected models, several experiments showed the task's simplicity required a redesigned Unet architecture with reduced complexity. This redesigned model yielded impressive results: the precision, recall, and Intersection over Union (IoU) scores were 0.91, 0.92, and 0.85, respectively. Our research could be improved upon by utilizing more diverse datasets, optimizing the model’s architecture, and conducting additional experiments with more advanced resources.
This article has been tagged with: