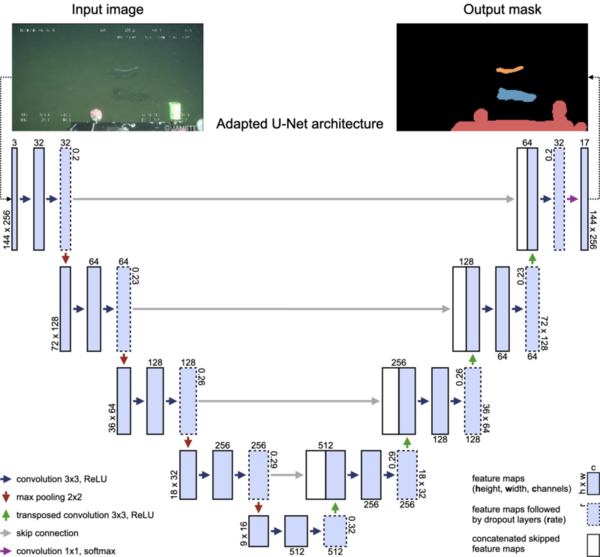
Plastic pollution in the ocean is a major global concern. Remotely Operated Vehicles (ROVs) have promise for removing debris from the ocean, but more research is needed to achieve full effectiveness of the ROV technology. Wahlig and Gonzales tackle this issue by developing a deep learning model to distinguish trash from the environment in ROV images.
Read More...