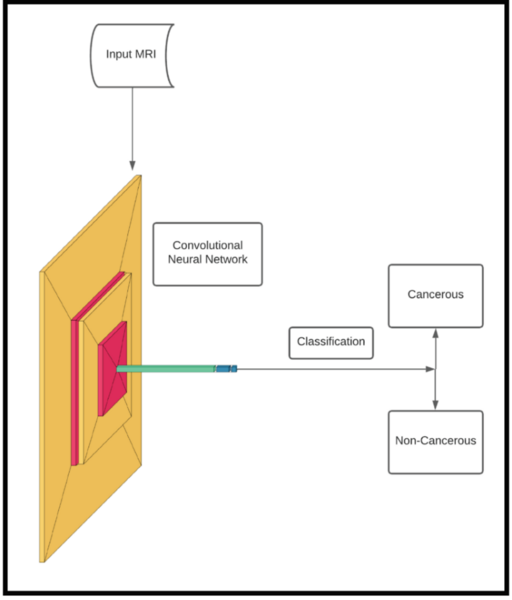
Using a convolution neural network, these authors show machine learning can clinically diagnose breast cancer with high accuracy.
Read More...Predicting the Instance of Breast Cancer within Patients using a Convolutional Neural Network
Using a convolution neural network, these authors show machine learning can clinically diagnose breast cancer with high accuracy.
Read More...A Novel Method for Auto-Suturing in Laparoscopic Robotic-Assisted Coronary Artery Bypass Graft (CABG) Anastomosis
Levy & Levy tackle the optimization of the coronary artery bypass graft, a life-saving surgical technique that treats artery blockage due to coronary heart disease. The authors develop a novel auto-suturing method that saves time, allows for an increased number of sutures, and improves graft quality over hand suturing. The authors also show that increasing the number of sutures from four to five with their new method significantly improves graft quality. These promising findings may help improve outcomes for patients undergoing surgery to treat coronary heart disease.
Read More...Recognition of animal body parts via supervised learning
The application of machine learning techniques has facilitated the automatic annotation of behavior in video sequences, offering a promising approach for ethological studies by reducing the manual effort required for annotating each video frame. Nevertheless, before solely relying on machine-generated annotations, it is essential to evaluate the accuracy of these annotations to ensure their reliability and applicability. While it is conventionally accepted that there cannot be a perfect annotation, the degree of error associated with machine-generated annotations should be commensurate with the error between different human annotators. We hypothesized that machine learning supervised with adequate human annotations would be able to accurately predict body parts from video sequences. Here, we conducted a comparative analysis of the quality of annotations generated by humans and machines for the body parts of sheep during treadmill walking. For human annotation, two annotators manually labeled six body parts of sheep in 300 frames. To generate machine annotations, we employed the state-of-the-art pose-estimating library, DeepLabCut, which was trained using the frames annotated by human annotators. As expected, the human annotations demonstrated high consistency between annotators. Notably, the machine learning algorithm also generated accurate predictions, with errors comparable to those between humans. We also observed that abnormal annotations with a high error could be revised by introducing Kalman Filtering, which interpolates the trajectory of body parts over the time series, enhancing robustness. Our results suggest that conventional transfer learning methods can generate behavior annotations as accurate as those made by humans, presenting great potential for further research.
Read More...Evaluating the clinical applicability of neural networks for meningioma tumor segmentation on 3D MRI
Authors emphasize the challenges of manual tumor segmentation and the potential of deep learning models to enhance accuracy by automatically analyzing MRI scans.
Read More...Floor level estimation using MEMS pressure sensors
The authors propose a method to help first responders find the location of a person within a high-rise building in densely populated areas.
Read More...Monitoring the formation of polyurethane foams with an infrared camera: Classroom activity
In this study, the authors utilize an infrared camera to visualize and investigate the exothermic reaction of polyurethane foam, which has many everyday uses including automotive seats, bedding, and insulation.
Read More...Unlocking robotic potential through modern organ segmentation
The authors looked at different models of semantic segmentation to determine which may be best used in the future for segmentation of CT scans to help diagnose certain conditions.
Read More...Vineyard vigilance: Harnessing deep learning for grapevine disease detection
Globally, the cultivation of 77.8 million tons of grapes each year underscores their significance in both diets and agriculture. However, grapevines face mounting threats from diseases such as black rot, Esca, and leaf blight. Traditional detection methods often lag, leading to reduced yields and poor fruit quality. To address this, authors used machine learning, specifically deep learning with Convolutional Neural Networks (CNNs), to enhance disease detection.
Read More...A novel CNN-based machine learning approach to identify skin cancers
In this study, the authors developed and assessed the accuracy of a machine learning algorithm to identify skin cancers using images of biopsies.
Read More...Building deep neural networks to detect candy from photos and estimate nutrient portfolio
The authors use pictures of candy wrappers and neural networks to improve nutritional accuracy of diet-tracking apps.
Read More...