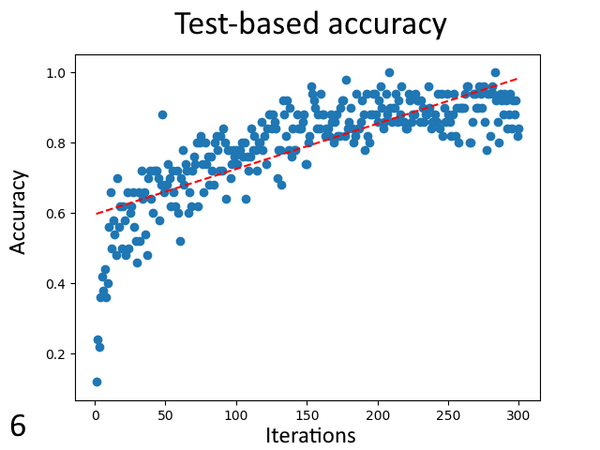
Neural networks are used throughout modern society to solve many problems commonly thought of as impossible for computers. Fountain and Rasmus designed a convolutional neural network and ran it with varying levels of training to see if consistent, accurate, and precise changes or patterns could be observed. They found that training introduced and strengthened patterns in the weights and visualizations, the patterns observed may not be consistent between all neural networks.
Read More...