Large Language Models are Good Translators
(1) Fontbonne Academy, (2) School of Mathematical Sciences, Capital Normal University
https://doi.org/10.59720/24-020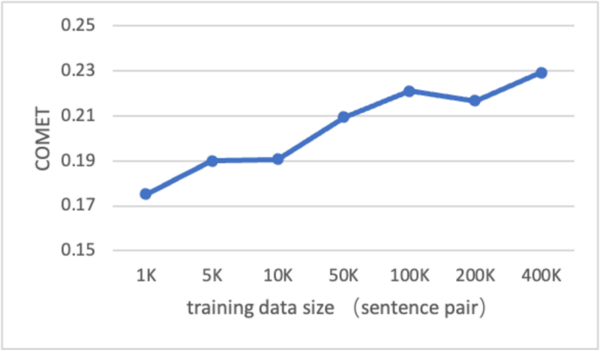
Machine translation, which uses computers to translate one language into another, is one of the most challenging tasks in artificial intelligence. During the last decade, neural machine translation (NMT), which builds translation models based on deep neural networks, has achieved significant improvement. However, NMT still faces several challenges. For example, the translation quality of an NMT system greatly depends on the amount of bilingual training data, which is expensive to acquire. Furthermore, it is difficult to incorporate external knowledge into an NMT system to obtain further improvement for a specific domain. Recently, large language models (LLMs) have demonstrated remarkable capabilities in language understanding and generation. This raises interesting questions about whether LLMs can be good translators and whether it is easy to adapt LLMs to new domains or to meet specific requirements. In this study, we hypothesized that LLMs can be adapted to perform translation by using prompts or fine-tuning and these adapted LLMs would outperform the conventional NMT model in four aspects: translation quality, interactive ability, knowledge incorporation ability, and domain adaptation. We compared GPT-4 and Google Translate, the representative LLM and NMT models, respectively, on the WMT 2019 (Fourth conference on machine translation) dataset. Experimental results showed that GPT-4 outperformed Google Translate in the above four aspects by exploiting appropriate prompts. Further experiments on Llama, an open-source LLM developed by Meta, showed that the translation quality of LLMs can be further improved by fine-tuning on limited language-related bilingual corpus, demonstrating strong adaptation abilities of LLMs.
This article has been tagged with: