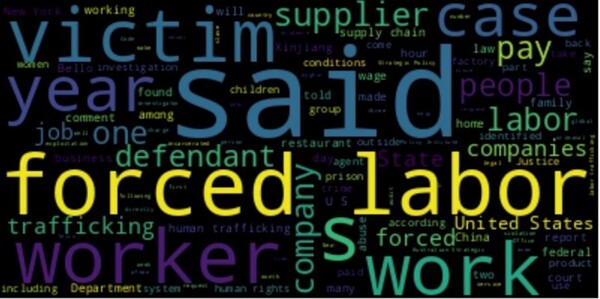
The authors use machine learning to develop an evidence-based detection tool for identifying human trafficking.
Read More...Uncovering the hidden trafficking trade with geographic data and natural language processing
The authors use machine learning to develop an evidence-based detection tool for identifying human trafficking.
Read More...Identifying shark species using an AlexNet CNN model
The challenge of accurately identifying shark species is crucial for biodiversity monitoring but is often hindered by time-consuming and labor-intensive manual methods. To address this, SharkNet, a CNN model based on AlexNet, achieved 93% accuracy in classifying shark species using a limited dataset of 1,400 images across 14 species. SharkNet offers a more efficient and reliable solution for marine biologists and conservationists in species identification and environmental monitoring.
Read More...Using Artificial Intelligence to Forecast Continuous Glucose Monitor(CGM) readings for Type One Diabetes
People with Type One diabetes often rely on Continuous Blood Glucose Monitors (CGMs) to track their blood glucose and manage their condition. Researchers are now working to help people with Type One diabetes more easily monitor their health by developing models that will future blood glucose levels based on CGM readings. Jalla and Ghanta tackle this issue by exploring the use of AI models to forecast blood glucose levels with CGM data.
Read More...Evaluating the clinical applicability of neural networks for meningioma tumor segmentation on 3D MRI
Authors emphasize the challenges of manual tumor segmentation and the potential of deep learning models to enhance accuracy by automatically analyzing MRI scans.
Read More...Evaluating the predicted eruption times of geysers in Yellowstone National Park
The authors compare the predicted versus actual geyser eruption times for the Old Faithful and Beehive Geysers at Yellowstone National Park.
Read More...Statistical models for identifying missing and unclear signs of the Indus script
This study utilizes machine learning models to predict missing and unclear signs from the Indus script, a writing system from an ancient civilization in the Indian subcontinent.
Read More...Examining the Growth of Methanotrophic Bacteria Immersed in Extremely Low-Frequency Electromagnetic Fields
Scientist are investigating the use of methane-consuming bacteria to aid the growing problem of rising greenhouse gas emissions. While previous studies claim that low-frequency electromagnetic fields can accelerate the growth rate of these bacteria, Chu et al. demonstrate that this fundamental ideology is not on the same wavelength with their data.
Read More...COVID-19 pandemic impact on emotional aspects of high school students
In this study, the impact of shutting down schools on the emotional aspects of high school students was analyzed using survey responses.
Read More...The Impact of Age on Post-Concussive Symptoms: A Comparative Study of Symptoms Related and Not Related to the Default Mode Network
The Default Mode Network (DMN) is a network of connected brain regions that are active when the brain is not focused on external tasks. Minor brain injuries, such as concussions, can affect this network and manifest symptoms. In this study, the authors examined correlations between DMN age and post-concussion symptoms in previously concussed individuals and healthy controls.
Read More...Functional Network Connectivity: Possible Biomarker for Autism Spectrum Disorders (ASD)
Autism spectrum disorder (ASD) is a complex neurodevelopmental disorder and is difficult to diagnose in young children. Here magnetoencephalography was used to compare the brain activity in patients with ASD to patients in a control group. The results show that patients with ASD have a high level of activity in different areas of the brain than those in the control group.
Read More...