Statistical models for identifying missing and unclear signs of the Indus script
(1) Dublin High School, Dublin, California, (2) Visiting professor of NLP at the Faculty of Computers and Information at Cairo University, Egypt
https://doi.org/10.59720/22-256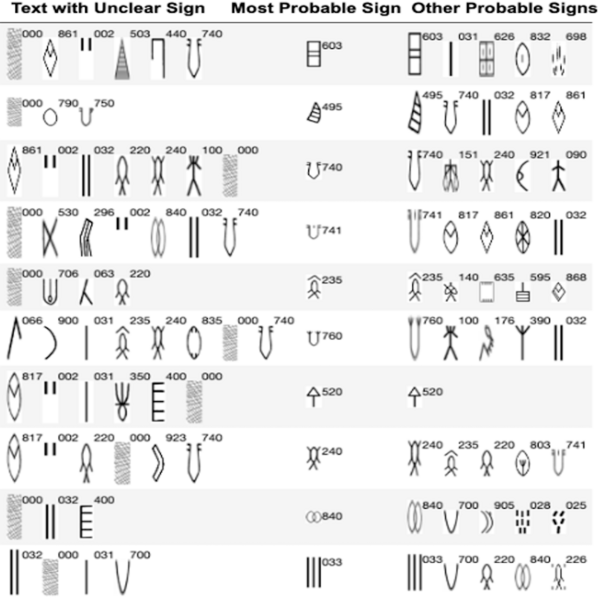
A writing system was developed between 2500 and 1800 BCE in the Indus Valley civilization in the Indian subcontinent and remains undeciphered. Indus script texts found so far in the archeological digs from this civilization are limited in number and include a lot of damaged artifacts with missing and unclear signs. Identifying the missing and unclear signs and extending the Indus text corpus will aid the researchers in deciphering this script. This work aimed to predict the missing and unclear signs using n-gram Markov chain models using the Interactive Corpus of Indus Texts (ICIT) text corpus. First, we analyzed patterns and concordances of the signs, pairs, triplets, and other n-grams and discovered the positional behavior of signs in the Indus texts. With that understanding, we built Markov chain language models based on n-grams, augmented with sign positional probabilities. Since signs could be missing in any location of the texts, we devised and implemented effective sign fill-in algorithms on top of these Markov chain models. Using the language models and the sign fill-in algorithms, we tuned our models and predicted single signs that were deliberately removed from complete texts with about 63% accuracy. Then we used the best model and our tuned parameters to predict missing and unclear single signs in about 100 texts. The statistical methods we described here improve our understanding of the Indus script. Filling in the missing signs makes the corpus more complete and helps contribute to the broader decipherment effort.
This article has been tagged with: