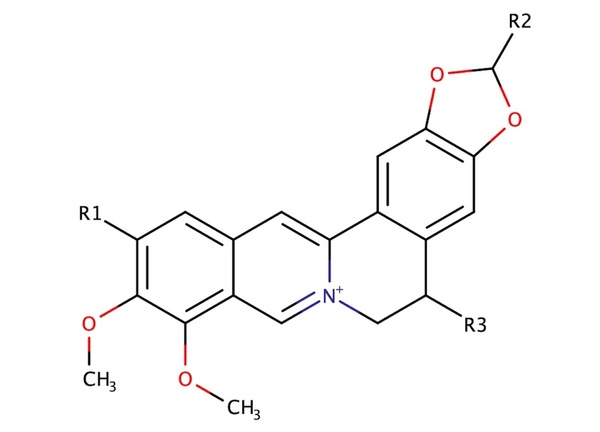
Berberine is a natural quaternary alkaloid that has anti-microbial and anti-cancer effects. This compound can bind to Guanine Quadruplex (G4) DNA secondary complexes to help inhibit cancer cell proliferation. In this study, the authors investigate whether incorporating large aromatic rings helps to stabilize berberine-G4 interactions.
Read More...