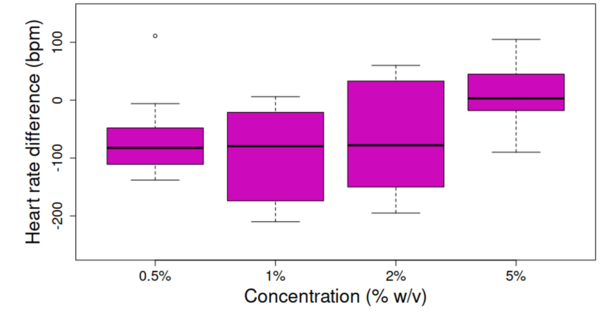
The authors test the effects of common food industry preservatives on the heart rate of the freshwater crustacean Daphnia magna.
Read More...The effect of common food preservatives on the heart rate of Daphnia magna
The authors test the effects of common food industry preservatives on the heart rate of the freshwater crustacean Daphnia magna.
Read More...Impact of carbon number and atom number on cc-pVTZ Hartree-Fock Energy and program runtime of alkanes
It's time-consuming to complete the calculations that are used to study nuclear reactions and energy. To uncover which computational chemistry tools are useful for this challenge, Pan, Vaiyakarnam, Li, and McMahan investigated whether the Python-based Simulations of Chemistry Framework’s Hartree-Fock (PySCF) method is an efficient and accurate way to assess alkane molecules.
Read More...Applying centrality analysis on a protein interaction network to predict colorectal cancer driver genes
In this article the authors created an interaction map of proteins involved in colorectal cancer to look for driver vs. non-driver genes. That is they wanted to see if they could determine what genes are more likely to drive the development and progression in colorectal cancer and which are present in altered states but not necessarily driving disease progression.
Read More...Aberrant response to dexamethasone suppression test associated with inflammatory response in MDD patients
Major depressive disorder (MDD) is a prevalent mood disorder. The direct causes and biological mechanisms of depression still elude understanding, though genetic factors have been implicated. This study looked to identify the mechanism behind the aberrant response to the dexamethasone suppression test (DST) displayed by MDD patients, in which they display a lack of cortisol suppression. Analysis revealed several pro-inflammatory genes that were significant and differentially expressed between affected and non-affected groups in response to the DST. Looking at ways to decrease the inflammatory response could have implications for treatment and may explain why some people treated for depression still display symptoms or may lead researchers to different classes of drugs for treatment.
Read More...Pruning replay buffer for efficient training of deep reinforcement learning
Reinforcement learning (RL) is a form of machine learning that can be harnessed to develop artificial intelligence by exposing the intelligence to multiple generations of data. The study demonstrates how reply buffer reward mechanics can inform the creation of new pruning methods to improve RL efficiency.
Read More...The effects of algaecides on Spirulina major and non-target organism Daphnia magna
Algal blooms pose a threat to ecosystems, but the methods used to combat these blooms might harm more than just the algae. Halepete, Graham, and Lowe-Schmahl demonstrate negative effects of anti-algae treatments on a cyanobacterium (Spirulina major), and the water fleas (Daphnia magna) that live alongside these cyanobacteria.
Read More...Modeling Hartree-Fock approximations of the Schrödinger Equation for multielectron atoms from Helium to Xenon using STO-nG basis sets
The energy of an atom is extremely useful in nuclear physics and reaction mechanism pathway determination but is challenging to compute. This work aimed to synthesize regression models for Pople Gaussian expansions of Slater-type Orbitals (STO-nG) atomic energy vs. atomic number scatter plots to allow for easy approximation of atomic energies without using computational chemistry methods. The data indicated that of the regressions, sinusoidal regressions most aptly modeled the scatter plots.
Read More...A novel approach for predicting Alzheimer’s disease using machine learning on DNA methylation in blood
Here, recognizing the difficulty associated with tracking the progression of dementia, the authors used machine learning models to predict between the presence of cognitive normalcy, mild cognitive impairment, and Alzheimer's Disease, based on blood DNA methylation levels, sex, and age. With four machine learning models and two dataset dimensionality reduction methods they achieved an accuracy of 53.33%.
Read More...Implementing machine learning algorithms on criminal databases to develop a criminal activity index
The authors look at using publicly available data and machine learning to see if they can develop a criminal activity index for counties within the state of California.
Read More...Simulation of cosmic rays in the presence of a magnetic field
In this study the authors looked the trajectories of cosmic rays moving through a dipole field. They found that the trajectories of cosmic rays are determined by a particle's energy and interaction with Earth's B field.
Read More...