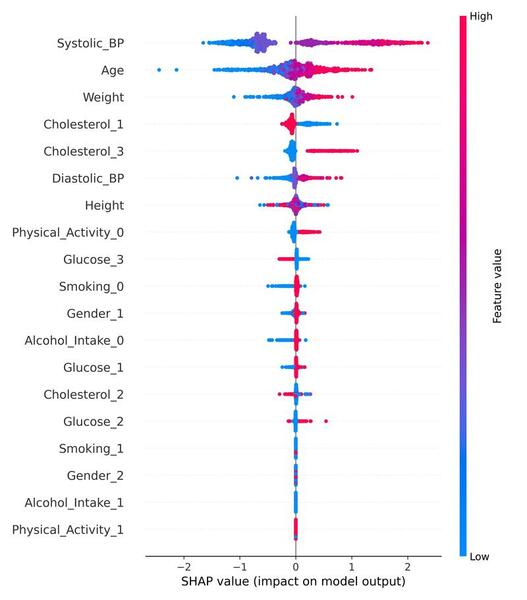
The authors test the effectiveness of machine learning to predict onset of cardiovascular disease.
Read More...Cardiovascular Disease Prediction Using Supervised Ensemble Machine Learning and Shapley Values
The authors test the effectiveness of machine learning to predict onset of cardiovascular disease.
Read More...Transfer Learning with Convolutional Neural Network-Based Models for Skin Cancer Classification
Skin cancer is a common and potentially deadly form of cancer. This study’s purpose was to develop an automated approach for early detection for skin cancer. We hypothesized that convolutional neural network-based models using transfer learning could accurately differentiate between benign and malignant moles using natural images of human skin.
Read More...Using machine learning to develop a global coral bleaching predictor
Coral bleaching is a fatal process that reduces coral diversity, leads to habitat loss for marine organisms, and is a symptom of climate change. This process occurs when corals expel their symbiotic dinoflagellates, algae that photosynthesize within coral tissue providing corals with glucose. Restoration efforts have attempted to repair damaged reefs; however, there are over 360,000 square miles of coral reefs worldwide, making it challenging to target conservation efforts. Thus, predicting the likelihood of bleaching in a certain region would make it easier to allocate resources for conservation efforts. We developed a machine learning model to predict global locations at risk for coral bleaching. Data obtained from the Biological and Chemical Oceanography Data Management Office consisted of various coral bleaching events and the parameters under which the bleaching occurred. Sea surface temperature, sea surface temperature anomalies, longitude, latitude, and coral depth below the surface were the features found to be most correlated to coral bleaching. Thirty-nine machine learning models were tested to determine which one most accurately used the parameters of interest to predict the percentage of corals that would be bleached. A random forest regressor model with an R-squared value of 0.25 and a root mean squared error value of 7.91 was determined to be the best model for predicting coral bleaching. In the end, the random model had a 96% accuracy in predicting the percentage of corals that would be bleached. This prediction system can make it easier for researchers and conservationists to identify coral bleaching hotspots and properly allocate resources to prevent or mitigate bleaching events.
Read More...Using economic indicators to create an empirical model of inflation
Here, seeking to understand the correlation of 50 of the most important economic indicators with inflation, the authors used a rolling linear regression to identify indicators with the most significant correlation with the Month over Month Consumer Price Index Seasonally Adjusted (CPI). Ultimately the concluded that the average gasoline price, U.S. import price index, and 5-year market expected inflation had the most significant correlation with the CPI.
Read More...Predicting baseball pitcher efficacy using physical pitch characteristics
Here, the authors sought to develop a new metric to evaluate the efficacy of baseball pitchers using machine learning models. They found that the frequency of balls, was the most predictive feature for their walks/hits allowed per inning (WHIP) metric. While their machine learning models did not identify a defining trait, such as high velocity, spin rate, or types of pitches, they found that consistently pitching within the strike zone resulted in significantly lower WHIPs.
Read More...Using broad health-related survey questions to predict the presence of coronary heart disease
Coronary heart disease (CHD) is the leading cause of death in the U.S., responsible for nearly 700,000 deaths in 2021, and is marked by artery clogging that can lead to heart attacks. Traditional prediction methods require expensive clinical tests, but a new study explores using machine learning on demographic, clinical, and behavioral survey data to predict CHD.
Read More...Enhancing marine debris identification with convolutional neural networks
Plastic pollution in the ocean is a major global concern. Remotely Operated Vehicles (ROVs) have promise for removing debris from the ocean, but more research is needed to achieve full effectiveness of the ROV technology. Wahlig and Gonzales tackle this issue by developing a deep learning model to distinguish trash from the environment in ROV images.
Read More...A natural language processing approach to skill identification in the job market
The authors looked at using machine learning to identify skills needed to apply for certain jobs, specifically looking at different techniques to parse apart the text. They found that Bidirectional Encoder Representation of Transforms (BERT) performed best.
Read More...An analysis of junior rower performance and how it is affected by rower's features
In this study, with consideration for the increasing participation of high school students in indoor rowing, the authors analyzed World Indoor Rowing Championship data. Statistical analysis revealed two key features that can determine the performance of a rower as well as increasing competitiveness in nearly all categories considered. They conclude by offering a 2000-meter ergometer time distribution that can help junior rowers assess their current performance relative to the world competition.
Read More...Evaluating the clinical applicability of neural networks for meningioma tumor segmentation on 3D MRI
Authors emphasize the challenges of manual tumor segmentation and the potential of deep learning models to enhance accuracy by automatically analyzing MRI scans.
Read More...