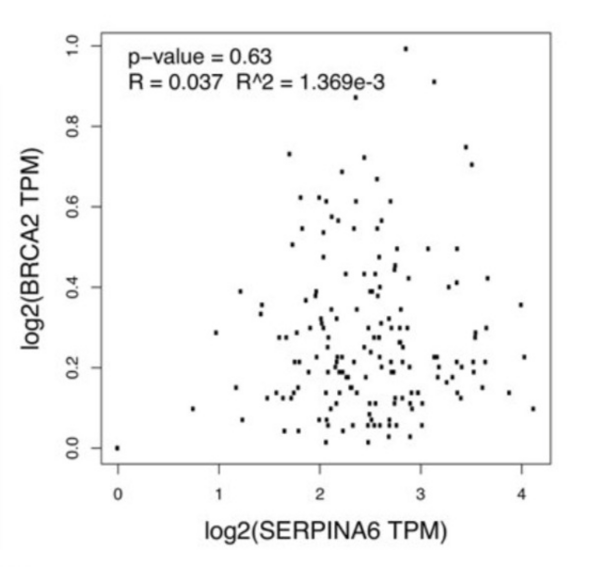
Pancreatic ductal adenocarcinoma (PDAC) is the most common form of pancreatic cancer, with early diagnosis and treatment challenges. When any of the genes KRAS, SMAD4, TP53, and BRCA2 are heavily mutated, they correlate with PDAC progression. Cellular stress, partly regulated by the gene SERPINA6, also correlates with PDAC progression. When SERPINA6 is highly expressed, corticosteroid-binding globulin inhibits the effect of the stress hormone cortisol. In this study, the authors explored whether there is an inverse correlation between the expression of SERPINA6 and PDAC-linked genes.
Read More...