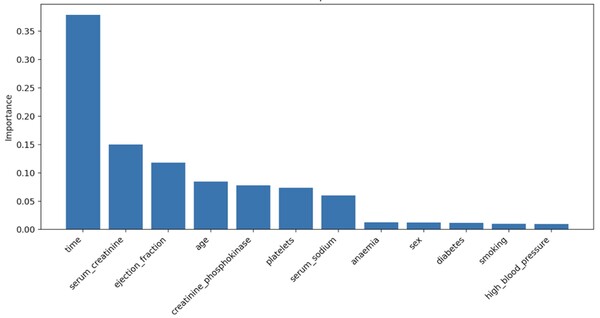
In 2021, over 20 million people died from cardiovascular diseases, highlighting the need for a deeper understanding of factors influencing heart failure outcomes. This study examined multiple variables affecting mortality after heart failure, using random forest models to identify time, serum creatinine, and ejection fraction as key predictors. These findings could contribute to personalized medicine, improving survival rates by tailoring treatment strategies for heart failure patients.
Read More...