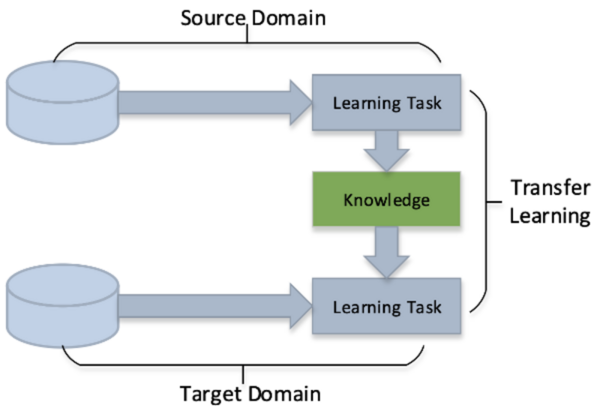
Osteosarcoma is a type of bone cancer that affects young adults and children. Early diagnosis of osteosarcoma is crucial to successful treatment. The current methods of diagnosis, which include imaging tests and biopsy, are time consuming and prone to human error. Hence, we used deep learning to extract patterns and detect osteosarcoma from histological images. We hypothesized that the combination of two different technologies (transfer learning and data augmentation) would improve the efficacy of osteosarcoma detection in histological images. The dataset used for the study consisted of histological images for osteosarcoma and was quite imbalanced as it contained very few images with tumors. Since transfer learning uses existing knowledge for the purpose of classification and detection, we hypothesized it would be proficient on such an imbalanced dataset. To further improve our learning, we used data augmentation to include variations in the dataset. We further evaluated the efficacy of different convolutional neural network models on this task. We obtained an accuracy of 91.18% using the transfer learning model MobileNetV2 as the base model with various geometric transformations, outperforming the state-of-the-art convolutional neural network based approach.
Read More...