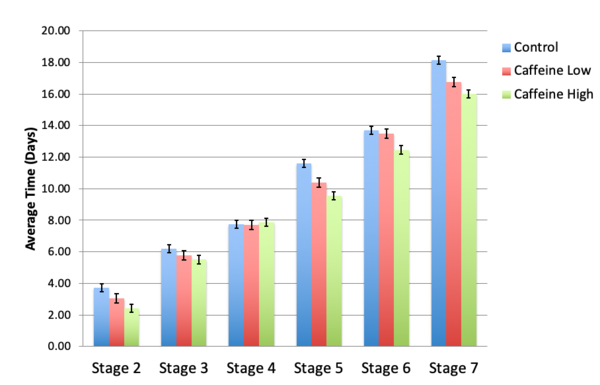
The degeneration of nerve cells in the brain can lead to pathologies such as Parkinson’s disease. It has been suggested that neurons in humans may regenerate. In this study, the effect of different doses of caffeine on regeneration was explored in the planeria model. Caffeine has been shown to enhance dopamine production, and dopamine is found in high concentrations in regenerating planeria tissues. Higher doses of caffeine accelerated planeria regeneration following decapitation, indicating a potential role for caffeine as a treatment to stimulate regeneration.
Read More...