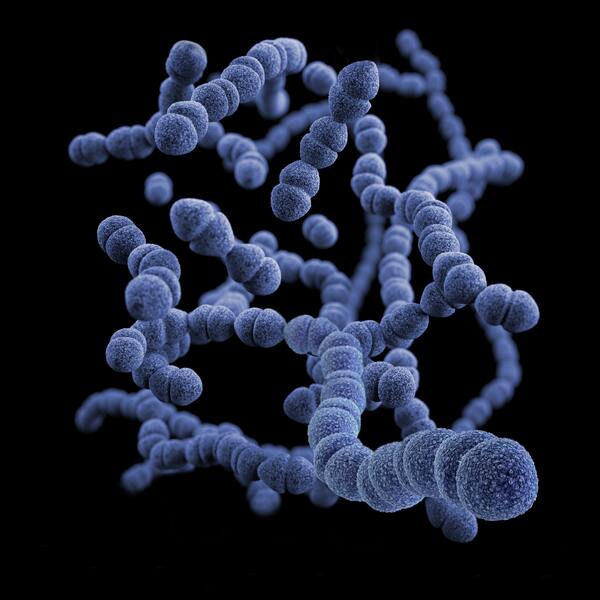
The authors investigated prophages present in Streptococcus bacteria that may increase their survival in different environments.
Read More...Distribution of prophages in the Streptococcus bacteria genus and their role in increasing host pathogenicity
The authors investigated prophages present in Streptococcus bacteria that may increase their survival in different environments.
Read More...Hybrid Quantum-Classical Generative Adversarial Network for synthesizing chemically feasible molecules
Current drug discovery processes can cost billions of dollars and usually take five to ten years. People have been researching and implementing various computational approaches to search for molecules and compounds from the chemical space, which can be on the order of 1060 molecules. One solution involves deep generative models, which are artificial intelligence models that learn from nonlinear data by modeling the probability distribution of chemical structures and creating similar data points from the trends it identifies. Aiming for faster runtime and greater robustness when analyzing high-dimensional data, we designed and implemented a Hybrid Quantum-Classical Generative Adversarial Network (QGAN) to synthesize molecules.
Read More...Solving a new NP-Complete problem that resembles image pattern recognition using deep learning
In this study, the authors tested the ability and accuracy of a neural net to identify patterns in complex number matrices.
Read More...Comparing the performance of lateral control algorithms on long rigid vehicles in urban environments
Here, seeking to better understand the control algorithms used in autonomous vehicles, the authors compared the Stanley and pure pursuit control algorithms along with a new version of each. Unexpectedly, they found that no control algorithm offered optimal performance, but rather resulted in tradeoffs between the various ideal results.
Read More...Association between nonpharmacological interventions and dementia: A retrospective cohort study
Here, the authors investigated the role of nonpharmacological interventions in preventing or delaying cognitive impairment in individuals with and without dementia. By using a retrospective case-control study of 22 participants across two senior centers in San Diego, they found no significant differences in self-reported activities. However, they found that their results reflected activity rather than the activity itself, suggesting the need for an alternative type of study.
Read More...Pediatric probiotic culture survival study in acidic pH using an in vitro model
In this study, the authors investigate the effects of acidity on the survival of commercial probiotic Lovebug bacterial strains.
Read More...The relationship between income inequality and maternal mortality for black and white mothers
In this study, the authors measure the relationship between the Gini coefficient, a common measure of income inequality, and Black and White maternal mortality rates by state and year.
Read More...Differential privacy in machine learning for traffic forecasting
In this paper, we measured the privacy budgets and utilities of different differentially private mechanisms combined with different machine learning models that forecast traffic congestion at future timestamps. We expected the ANNs combined with the Staircase mechanism to perform the best with every value in the privacy budget range, especially with the medium high values of the privacy budget. In this study, we used the Autoregressive Integrated Moving Average (ARIMA) and neural network models to forecast and then added differentially private Laplacian, Gaussian, and Staircase noise to our datasets. We tested two real traffic congestion datasets, experimented with the different models, and examined their utility for different privacy budgets. We found that a favorable combination for this application was neural networks with the Staircase mechanism. Our findings identify the optimal models when dealing with tricky time series forecasting and can be used in non-traffic applications like disease tracking and population growth.
Read More...The novel function of PMS2 mutation on ovarian cancer proliferation
With disruption of DNA repair pathways pertinent to the timeline of cancer, thorough evaluation of mutations relevant to DNA repair proteins is crucial within cancer research. One such mutation includes S815L PMS2 - a mutation that results in significant decrease of DNA repair function by PMS2 protein. While mutation of PMS2 is associated with significantly increased colorectal and endometrial cancer risk, much work is left to do to establish the functional effects of the S815L PMS2 mutation in ovarian cancer progression. In this article, researchers contribute to this essential area of research by uncovering the tumor-progressive effects of the S815L PMS2 mutation in the context of ovarian cancer cell lines.
Read More...Linear and non-linear summation of responses to visual and olfactory cues in male Drosophila melanogaster
In this study, the authors investigate whether phototaxis and odortaxis in Drosophila melanogaster occurs through linear summation of cues including light and attractive odorants.
Read More...