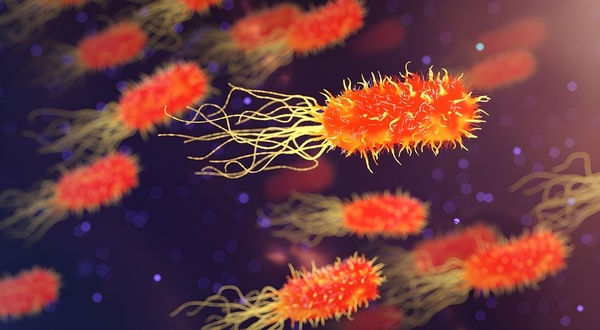
The independent effects of metastasis-promoting gene CD151 in the process of metastasis are not known. This study aimed to isolate CD151 to discover what its role in metastasis would be uninfluenced by potential interactions with other components and pathways in human cells. Results showed that CD151 significantly increased the adhesion of the cells and decreased their motility. Thus, it may be that CD151 is upregulated in cancer cells for the last step of metastasis, and it increases the chances of success of metastasis by aiding in implantation of the cancer cells. Targeting CD151 in chemotherapeutic modalities could therefore potentially slow or prevent metastasis.
Read More...